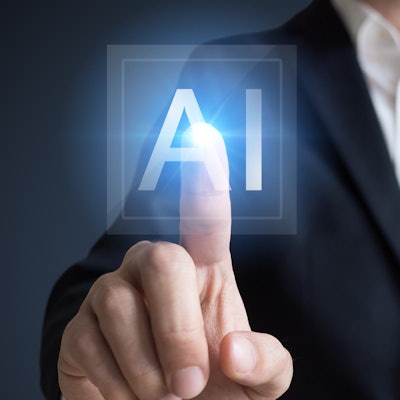
Pretraining deep-learning models with YouTube video clips can significantly increase the performance of these algorithms in certain radiology applications and help to overcome the challenge of assembling adequate training datasets, according to research published online March 3 in Scientific Reports.
A team of researchers from Stanford University used a dataset of over 500,000 annotated YouTube video clips of natural videos to pretrain AppendiXNet, a deep-learning model for detecting appendicitis on CT exams. The algorithm was subsequently able to produce a high discriminative performance for appendicitis despite being trained on less than 500 CT exams.
"Our work shows the utility of pretraining with videos with a small clinical dataset size and could generalize to other challenging cross-sectional medical imaging applications with limited datasets," wrote the authors. Pranav Rajpurkar; Allison Park; Jeremy Irvin; Andrew Ng, PhD; and Dr. Bhavik Patel all contributed equally to the research.
It can be challenging to build sufficient image datasets to adequately train artificial intelligence (AI) algorithms for specific radiology use cases. Pretraining of models using large datasets of natural images, such as ImageNet, has been helpful for tackling 2D medical imaging tasks, but it doesn't apply as easily to the 3D data produced by cross-sectional imaging devices, according to the researchers.
In their retrospective study, they sought to determine if pretraining a model with a large dataset of natural videos would yield better results than training the model from scratch. Their algorithm -- AppendiXNet -- is an 18-layer 3D convolutional neural network (CNN) that analyzes contrast-enhanced CT scans and provides a probability indicating the presence or absence of appendicitis.
The researchers compared the performance of AppendiXNet with and without pretraining on Kinetics, an open-source collection of approximately 500,000 10-second YouTube video clips that are annotated for one of 600 human action classes. A dataset of 646 contrast-enhanced CT exams of the abdomen and pelvis in patients who had presented to the emergency room with abdominal pain at their institution was used to train AppendiXNet.
Of the CT dataset, 438 exams were placed in a training set and 106 were used in a development set. The remaining 102 studies were set aside for the test set. Of the cases in the development and test sets, approximately half were appendicitis exams and half were nonappendicitis cases.
Impact of YouTube training on AI detecting appendicitis on CT exams | ||
AppendiXNet without pretraining on YouTube videos | AppendiXNet with pretraining on YouTube videos | |
Area under the curve (AUC) | 0.724 | 0.810 |
This difference in AUC was statistically significant (p = 0.025). The performance of the pretrained AppendiXNet is also comparable to previously published 3D CNN models for detection of emergent findings on CT, according to the researchers.
In discussing the results, the authors noted that interpretation of cross-sectional medical images often requires 3D context, involving continuous scrolling of contiguous 2D slices of a patient's scan.
"Thus, the presence of abnormalities or pathologies become apparent using spatiotemporally related information from multiple slices," they wrote. "Moreover, visual representations necessary for complex spatiotemporal understanding tasks may not be adequately learned using static images; instead feature representations can be learned using videos, resulting in improved model performance."