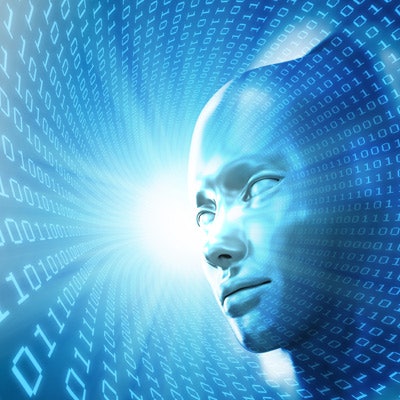
An artificial intelligence (AI) algorithm can categorize indeterminate lung nodules on CT better than existing clinical risk models, according to research published online April 24 in the American Journal of Respiratory and Critical Care Medicine.
Trained using low-dose CT (LDCT) images from the National Lung Screening Trial (NLST), a deep-learning algorithm developed by a multinational team of researchers enabled at least one-third more of the cancers and benign nodules on two different external validation test sets to be accurately reclassified than a traditional risk model.
"These results suggest the potential clinical utility of this deep-learning algorithm to revise the probability of cancer among [indeterminate pulmonary nodules] aiming to decrease invasive procedures and shorten time to diagnosis," said first author Dr. Pierre Massion of Vanderbilt University in a statement.
A number of guidelines exist for managing indeterminate pulmonary nodules. However, adherence to guidelines can be variable, and patient cases can be classified subjectively, according to the authors. As a result, the researchers developed Lung Cancer Prediction Convolutional Neural Network (LCP-CNN) in an effort to create an unbiased prediction tool for clinicians. After analyzing the images, LCP-CNN outputs a likelihood of malignancy score ranging from 0%-100%.
Training and internal validation was performed with an NLST dataset of LDCT exams that included 14,761 benign nodules from 5,972 patients and 932 malignant nodules from 575 patients. The researchers then compared the algorithm's performance with the traditional Mayo Clinic risk prediction model on two external validation datasets from Vanderbilt University Medical Center (VUMC) and Oxford University Hospital (OUH) NHS Foundation Trust in the U.K.
The VUMC dataset was a prospectively gathered collection from 116 patients who had been referred to their lung nodule clinic with incidental pulmonary nodules. The 116 nodules in these patients included 52 benign and 64 malignant nodules.
A retrospectively collected set of incidental pulmonary nodules, the OUH dataset included 463 nodules from 427 patients. This set included 63 malignant nodules from 62 patients.
The AI algorithm produced a higher area under the curve (AUC) on the VUMC set (91.9% versus 81.9%) and on the OUH test set (83.5% versus 78.1%). The improvement in the VUMC test set was statistically significant (p = 0.018), but not for the OUH set (p = 0.082).
To find out how many more cases the AI algorithm could reclassify in comparison with the Mayo model, the researchers calculated the net reclassification benefit for cancers and benign nodules.
Net reclassification benefit compared with Mayo risk prediction model | ||
AI algorithm as a rule-in test (> 65% malignancy threshold for a high-risk category | AI algorithm as a rule-out test (< 5% malignancy threshold for low-risk category) | |
VUMC test set | 34% | 33% |
OUH test set | 30% | 58% |
In addition, "The LCP-CNN compared to traditional risk prediction models was associated with an improved accuracy in the predicted likelihood of disease at each threshold of management and in our external validation cohorts," the authors wrote.
The researchers noted that their model is intended to be improved over time as data collections are added and structured curation efforts continue.
"While more stringent clinical validations on additional (external and independent) datasets are needed, our results suggest that it may be possible to address a major problem in the management of individuals presenting with indeterminate pulmonary nodules using a machine learning derived prediction model," they wrote.