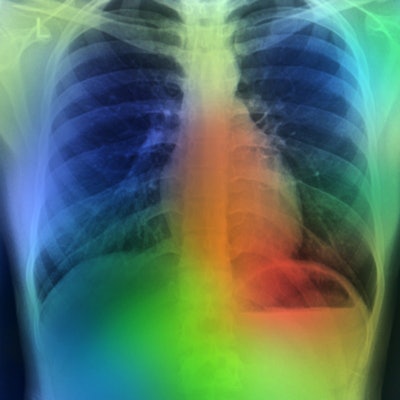
A peer review process that's driven by artificial intelligence (AI) can identify lung nodules that were overlooked by radiologists on CT exams, potentially enabling early detection of cancers that would have otherwise been missed, according to a Monday talk at the Conference on Machine Intelligence in Medical Imaging (C-MIMI).
After installing a lung nodule detection AI platform, Sutter Health in California found that the software enabled discovery of missed nodules in 0.6% of exams it processed over a nine-month period. Many of these nodules were deemed to be significant enough to change patient care.
"We continue to see significant findings throughout the course of the study so far," said Dr. Jason Wiesner, diagnostic imaging service line medical director. He shared his institution's experience during a scientific session at C-MIMI 2020, which is being held by the Society for Imaging Informatics in Medicine (SIIM).
Early detection
Lung cancer makes up approximately 25% of cancer deaths in the U.S., and it has a lower five-year survival rate than other cancers. Lung nodules are often found incidentally on CT and have been shown to have about a 4% chance of malignancy; early detection of small malignant lung nodules can improve survival by approximately five times, according to Wiesner.
However, the existing peer review process for detecting medical errors isn't sufficient for discovering lung nodules that were missed by radiologists, he said.
"Traditional peer review, which, let's be honest, is what most departments are using nowadays, is severely limited by the small proportion of studies being peer reviewed," Wiesner said.
The literature suggests that somewhere between 3% to 5% of all studies should be reviewed by peers. However, this approach involves a random selection of cases, Wiesner said.
"And there's no real clear follow-up protocols for patients when a nodule is detected or some other error or discrepancy is detected on that peer review," he said. "I think where we need to go is peer reviewing a larger study selection and more of our population and have the opportunity to do a curated or more focused review of a particular radiologist, of a section, of a modality such as CT, or an indication such as lung nodules. And we can get there with AI technology."
AI-driven peer review
Sutter Health embarked on an AI-driven peer review project by deploying a commercial AI platform (Ferrum Health) to identify lung nodules that may have been overlooked on prior interpretation by radiologists. Designed to serve as an adjunct to peer review, the software uses two classifiers that work in tandem: a 3D convolutional neural network to analyze CT images and a natural language processing algorithm to find radiology reports that include a positive mention of a lung nodule.
At their institution, all CT scans with lung tissue are sent to the software, which analyzes the images for the presence of lung tissue and then lung nodules. In addition, the final signed radiology reports are analyzed for mentions of a lung nodule or mass. Studies with a discrepancy between the images and the final reports are then flagged for further review by a group of fellowship-trained radiologists, according to Wiesner.
After the expert review, the study is then set back to the original dictating radiologist, who can then confirm that there was a nodule, issue an addendum to that report, and then prescribe any follow-up treatments, such as biopsy, he said.
The researchers assessed the software in an evaluation period between August 2019 and April 2020 and reported the following results:
- Studies processed by software: 33,185
- Studies flagged for review by software: 1,222 (3.7%)
- Missed nodules: 210 (17% of flagged studies and 0.6% of all studies)
- Significant nodules that changed patient care: 123
Of the missed nodules, 42% were from abdomen/pelvis CT; 33% were from chest CT exams; 14% were on chest, abdomen, and pelvis CT exams; and 10% were found on other CT exams, Wiesner said.
Improving discrepancy detection
In a traditional peer review process that would review only 1%-3% of CT scans and a 3%-5% estimated radiology error rate, there is a 0.08% probability a discrepancy would be detected. In nine months, 210 misses were caught through AI-enabled peer review of CT scans with lung tissue. As a result, the AI-enabled peer review process could improve discrepancy detection by approximately eight times, according to Wiesner.
Sutter Health is now in the process of implementing the software across its health system, Wiesner said. Once it's deployed system-wide, 490,000 CT scans could be processed over a five-year period at Sutter Health. Assuming the same 0.6% rate of missed nodules found during the trial study, 3,000 missed nodules would be discovered and 120 of these would be malignancies that would be detected as a result of this project, he said.
Although Sutter Health is currently just using the software to search for pulmonary nodules on CT scans, Wiesner is hopeful that they can use the technology in the future for multiple indications, such as looking for masses or calcifications in mammograms, pneumothorax on chest x-rays, etc. In addition, Wiesner plans to use the software to assist in learning, perform further analysis of factors that lead to missed nodules, and perhaps even to support optimization of radiation dose, he said.