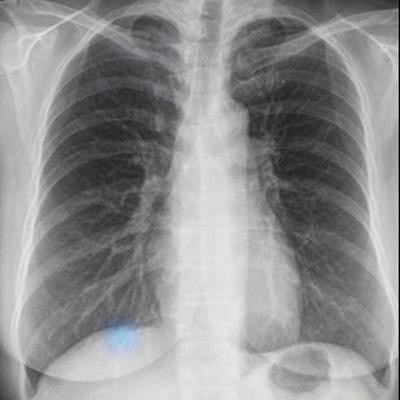
An artificial intelligence (AI) algorithm can catch lung cancers that were initially missed by radiologists on chest radiographs and improve radiologist performance when used as a second reader, according to research published online December 10 in Radiology: Cardiothoracic Imaging.
In testing on an enriched dataset, a team of researchers led by Dr. Ju Gang Nam of Seoul National University Hospital in South Korea found that a commercial deep-learning algorithm yielded significantly higher sensitivity and specificity than four thoracic radiologists. When used by the radiologists as a second reader, the model also significantly improved their detection performance.
Although computer-aided diagnosis (CAD) software has previously been developed to limit human errors in chest radiography, these applications have not been widely adopted in clinical practice due to performance issues, according to the researchers.
To see if a deep-learning algorithm could yield better results, they compared the performance of version 1.0.1.1 of the Insight CXR software (Lunit) with that of four thoracic radiologists. The study included an enriched dataset with 50 normal chest radiographs and 168 posteroanterior chest radiographs with 187 lung cancers at a mean size of 2.3 ± 1.2 cm.
The radiologists, who had nine, six, six, and five years of experience, respectively, read the radiographs first on their own and then with the help of the AI.
Performance of AI algorithm and thoracic radiologists in detecting lung cancer on chest x-rays | |||
AI algorithm | Thoracic radiologists alone (pooled performance) | Thoracic radiologists with help from AI (pooled performance) | |
Sensitivity (per radiograph) | 70% | 47% | 53% |
Specificity (per radiograph) | 94% | 78% | 82% |
Sensitivity (per lesion localization) | 54% | 44% | 50% |
False-positive rate (per lesion localization) | 21% | 19% | 19% |
Unlike previously reported CAD systems that were shown to yield frequent false-positive findings, the AI software produced significantly higher specificity (p = 0.01) than that of the pooled radiologists, according to the researchers. What's more, the radiologists experienced significantly higher sensitivity (p < 0.001) and specificity (p < 0.01) after they had assistance from the algorithm, according to the researchers.
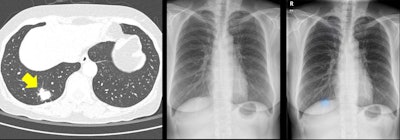
Although their performance improved after using the algorithm, the radiologists could not reach the model's standalone performance level, according to the authors. The readers were likely to miss retrocardiac and retrodiaphragmatic nodules. At lower lung fields, they also neglected more true nodules, regarding them as nipple or vascular shadow, the researchers noted.
"Because the algorithm takes pixel values of the image, it may recognize abnormalities in areas of soft-tissue density better than do human readers," they wrote. "In addition, readers accepted larger nodules and showed limited acceptance rate for subcentimeter nodules."
Assuming that further vetting of the algorithm demonstrates its worth, financial considerations will be the final challenge to overcome, according to Dr. Charles White of the University of Maryland School of Medicine.
"Although CAD-based systems did not achieve wide clinical use, in part because of performance issues as noted by the authors, the lack of reimbursement in most locales was certainly a contributory factor," White wrote in an accompanying commentary. "If this algorithm indeed proves far superior to the capability of readers with low false-positive results, it would provide a clearer pathway to investing in impetus for further research to determine the precise value of this and other deep learning-based algorithms for nodule detection at chest radiography."