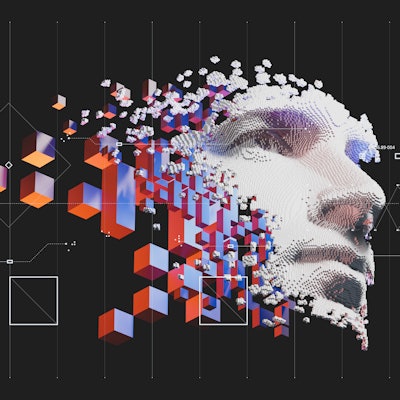
An artificial intelligence (AI) algorithm was able to help accurately detect traumatic brain injury (TBI) on head CT exams, as well as assess the type of injury, quantify lesion burden, and measure lesion progression, U.K. researchers reported in an article published online May 14 in Lancet Digital Health.
After training a convolutional neural network (CNN), a team of researchers led by Miguel Monteiro of the Imperial College London and Virginia Newcombe, PhD, of the University of Cambridge in the U.K. found that their deep-learning algorithm could accurately identify, segment, and quantify multiple classes of TBI hemorrhagic lesions as well as perilesional edema.
"These volumetric lesion estimates allow clinically relevant quantification of lesion burden and progression, with potential applications for personalized treatment strategies and clinical research in TBI," the authors wrote.
Although CT is the most commonly used imaging modality for TBI, these studies require experts to interpret and aren't typically utilized to generate quantitative measurements, according to the researchers. As a result, they sought to develop a deep-learning algorithm in hopes of reliably and efficiently quantifying and detecting different TBI lesion types.
The team trained and tested their algorithm using data from patients from 60 centers across Europe who were recruited between December 2014 and December 2017.
After initial training and validation of a CNN using manual segmentations, the researchers used the CNN to automatically segment a new dataset of scans. This dataset, which was corrected and reviewed by experts for accuracy, was then utilized to train the final CNN to perform segmentation of multiple classes of lesions on head CT images.
The researchers then assessed the algorithm's performance on a test set of 655 scans.
Mean difference between AI algorithm and manual measurements for TBI lesions | |
Intraparenchymal hemorrhage | 0.86 mL |
Extra-axial hemorrhage | 1.83 mL |
Intraventricular hemorrhage | 0.07 mL |
Perilesional edema | 2.09 mL |
The researchers also performed external validation of the algorithm using the CQ500 dataset. In this test, the algorithm yielded an area under the curve (AUC) of 0.89 for any lesion, 0.87 for intraparenchymal hemorrhage, 0.89 for the intraparenchymal hemorrhage, 0.89 for extra-axial hemorrhage, and 0.89 for perilesional edema.
The results show that these types of lesions can be detected and measured by the CNN with a high level of accuracy, according to the researchers.
Future work will need to focus on incorporating these types of algorithms into clinical practice and include rigorous assessment, according to the researchers.
"Such algorithms will find clear research applications, and, if adequately validated, may be used to help facilitate radiology workflows by flagging scans that require urgent attention, aid reporting in resource-constrained environments, and detect pathoanatomically relevant features for prognostication and a better understanding of lesion progression," they wrote.
The researchers said they have made their algorithm available for free in order to facilitate future research.