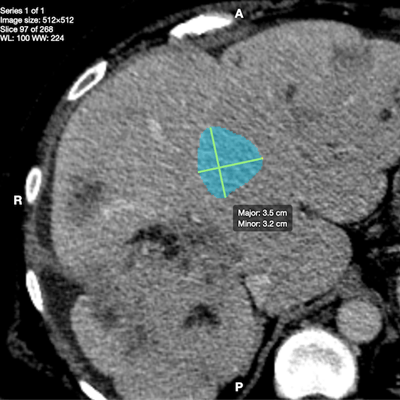
Artificial intelligence (AI) software can significantly enhance assessment of cancer treatment response on serial CT exams by reducing errors, speeding up interpretations, and decreasing interobserver variability, according to research presented May 29 at the online American Society of Clinical Oncology (ASCO) meeting.
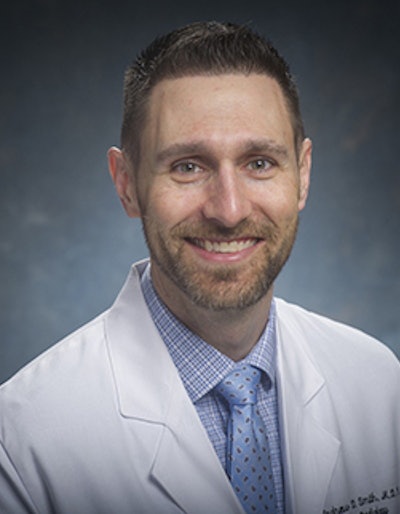
In a multi-institutional study, a team of researchers led by Dr. Andrew Smith, PhD, of the University of Alabama at Birmingham (UAB) found that the AI software led to a 99% reduction in major errors, 25% improved accuracy, and a 45% improvement in interreader agreement. What's more, AI-assisted interpretations were nearly twice as fast.
Benefits of the technology include "improved workflow for the radiologists, who are now more than ever burdened with complex imaging studies and increased incidence of burnout, and for our clinical colleagues, a clear and concise longitudinal report," said Smith in a statement from UAB. "This is a monumental improvement to the current standard of care and will in fact set a new standard."
Smith is co-director of AI in UAB's department of radiology and director of the Tumor Metrics Lab at O'Neal Cancer Center. He is also CEO of AI Metrics, a UAB spin-off that's now raising a seed round of capital. The software used in the study is a cancer-specific implementation of the company's medical AI software platform that was trained on 15,000 images.
The researchers retrospectively tested the AI software on body CT images from 120 consecutive patients who had received systemic therapy for advanced cancer and who had multiple serial imaging exams. After the software is activated by the radiologist, it measures the tumors, automatically labels their anatomic location, and automatically categorizes tumor response per Response Evaluation Criteria in Solid Tumors (RECIST) 1.1 calculations. It also produces AI-assisted reports that include a graph, table, and key images.
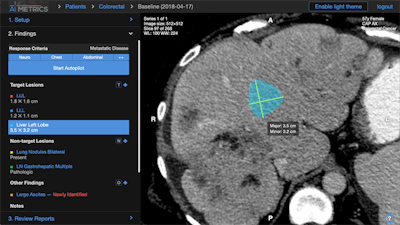
All exams were also independently evaluated in triplicate by 24 radiologists, producing a total test sample of 360 cases. They first read the exams using the current practice method -- dictating text-based reports and separately categorizing treatment response. They then interpreted the studies with help from the AI software. Twenty oncologic providers then assessed the accuracy of the reports.
Performance of AI for assessing treatment response on serial body CT exams | ||
Radiologists without AI | Radiologists with AI | |
Major errors (incorrect measurements, erroneous language in reports, or misidentifying tumor location) | 99/360 (27.5%) | 1/360 (0.3%) |
Average interpretation time | 18.7 minutes | 9.8 minutes |
Total interreader agreement on final treatment response categorization | 62/120 (52%) | 90/120 (75%) |
Of the 24 radiologists in the study, 96% preferred the AI-assisted software. What's more, the 20 oncologic providers all preferred the AI-assisted reports.
"I think that having this software could save lives, though we don't yet have that kind of data," Smith said.
The researchers said they have continued to improve the algorithm since the study. The model has been retrained on 55,000 tumors, with plans to reach 100,000 in the coming months, according to Smith.
In addition, they are now pursuing a grant from the U.S. National Institutes of Health in order to apply the technology to cancer screening and early detection and management of cancer.
"This technology applies to all solid cancers imaged on CT and MRI," Smith said. "We can apply this technology to many other stages of cancer."