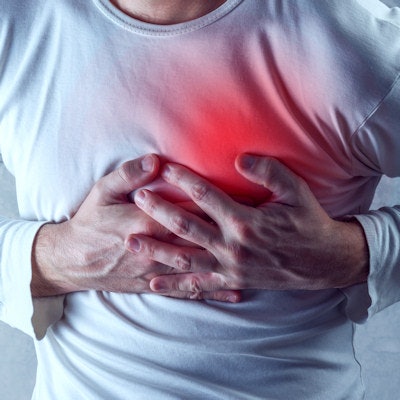
A machine-learning algorithm for analysis of coronary CT angiography (CCTA) exams was able to predict major adverse cardiac events at a higher level of accuracy than other traditional risk scores and risk factors, according to a presentation on Tuesday morning at the virtual RSNA 2020 meeting.
In a retrospective study, researchers led by Dr. Christian Tesche of the Medical University of South Carolina found that their machine-learning model could improve risk stratification for major cardiac adverse events compared with conventional risk scores and clinical information. It also outperformed conventional regression analysis.
"Machine learning may improve the integration of patient information to improve risk stratification," Tesche said.
CCTA-based risk scores mostly reflect the coronary plaque burden by the location, extent, and severity of coronary artery disease (CAD), according to Tesche. Previous studies have shown that these risk scores have prognostic value, yielding superior outcome predictions to traditional clinical risk scores.
As machine learning can yield improved time efficiency and diagnostic accuracy for optimizing predictions based on various input features, the researchers sought to use the technology to evaluate the long-term prognostic value of CCTA-derived plaque measures and clinical parameters on major adverse cardiac events, Tesche said.
The researchers retrospectively analyzed a dataset of 361 patients who were suspected to have CAD and who received a CCTA exam. Next, they recorded the occurrence of major adverse cardiac events more than 90 days after the CCTA study. These events included cardiac death as well as unstable angina leading to coronary revascularization with more than six weeks between CCTA and revascularization procedure. Of the 361 patients, 31 (8.6%) had a major adverse cardiac event over the median follow-up period of 5.4 years.
The authors then assessed several CCTA-derived plaque measures, including low-attenuation plaque, napkin-ring sign, spotty calcifications, remodeling index, segment stenosis score, and segment involvement score. In addition, they obtained cardiovascular risk factors and the Framingham risk score from medical records. A total of 28 of these variables were then provided to a machine-learning model that's based on RUSBoost, an ensemble classification algorithm that utilizes decision trees.
"RUSBoost is particularly well-suited for imbalanced datasets which are typically observed in the context of [major adverse cardiac event] prediction," Tesche said.
The researchers then compared the performance of the algorithm for predicting major adverse cardiac events with that of conventional regression analysis, as well as individual variables such as conventional CCTA risk scores, plaque measures, and clinical information.
Machine-learning performance for predicting major adverse cardiac events | ||||||
Obstructive CAD | Cardiovascular risk factors | Agatston calcium score | Segment stenosis score | High-risk plaque factors | Machine-learning model | |
Area under the curve | 0.79 | 0.83 | 0.84 | 0.89 | 0.89 | 0.96 |
Furthermore, the algorithm also outperformed conventional regression analysis, which produced an area under the curve of 0.92. That difference was also statistically significant (p = 0.024).
Tesche acknowledged a number of limitations of the study, including the lack of an external validation cohort. In addition, its retrospective design also could induce a selection bias, he said. As patient follow-up was performed using electronic medical record searches and patient phone calls, major adverse cardiac events may also be underreported.