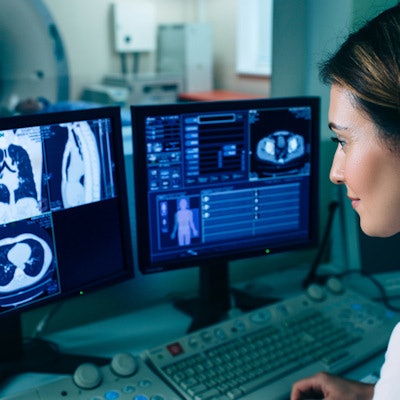
An artificial intelligence (AI) deep learning-based risk prediction model trained on chest x-rays and medical record data improves assessment of patients' eligibility for CT lung cancer screening, according to a review published December 15 in the American Journal of Roentgenology.
In a "Beyond the AJR" review of research published in November in the Annals of Internal Medicine, researchers from Stanford University lauded the model, called CXR-LC, noting that it performed better for identifying patients eligible for lung cancer screening than criteria from the U.S. Centers for Medicare and Medicaid (CMS).
"CXR-LC significantly outperformed CMS screening eligibility for 12-year incidence lung cancer," wrote review coauthors Dr. Bhavik Patel and Dr. Curtis Langlotz, PhD.
The CMS's current eligibility criteria for CT lung cancer screening misses most lung cancers, Patel and Langlotz noted. To address this problem, a team led by Dr. Michael Lu of the Massachusetts General Hospital Cardiovascular Imaging Center (CIRC) developed a multimodal deep-learning risk prediction model that uses 32 features from chest x-rays and 32 from medical record data (such as age, gender, and current smoking status) to predict lung cancer incidence at six and 12 years after assessment and 12-year lung cancer deaths (Annals of Internal Medicine, November 3, 2020, Volume 173:9, pp. 704-713).
Lu and colleagues trained and validated the model on 52,320 chest x-rays taken from the Prostate, Lung, Colorectal, and Ovarian (PLCO) trial; it was also validated on 5,493 x-rays from the National Lung Cancer Screening Trial (NLST). The researchers compared the model's performance with that of CMS eligibility screening and with a logistic regression risk model based on PLCO data.
The investigators found that the model outperformed CMS lung cancer screening eligibility criteria for 12-year incidence of the disease and performed similarly to the risk model based on the PLCO data, CMS-eligible smokers in the PLCO cohort, and CMS-ineligible patients. For the NLST dataset, CXR-LC performed comparably to the PLCO risk model. They also found similar results for six-year incidence of lung cancer.
As for 12-year incidence of lung cancer deaths, CXR-LC bested CMS eligibility criteria and performed comparably to the PLCO risk model across all patient subsets and the NLST cohort. It missed 30.7% fewer lung cancers at 12-year diagnosis than the CMS eligibility criteria.
CMS vs. CXR-LC for identifying patients eligible for lung cancer screening | ||
Measure | CMS eligibility criteria | CXR-LC deep-learning risk prediction model |
12-year incident lung cancer* | 0.63 | 0.75 |
12-year lung cancer deaths* | 0.64 | 0.76 |
The research "highlights the added value imaging and machine learning may play in a patient's care beyond conventional image interpretation," according to Patel and Langlotz.
"[Lu's] study shows the power of deep learning methods and highlights an important recent trend in machine learning in the use of both tabular electronic medical record data and imaging data for multimodal algorithm development," the two concluded.