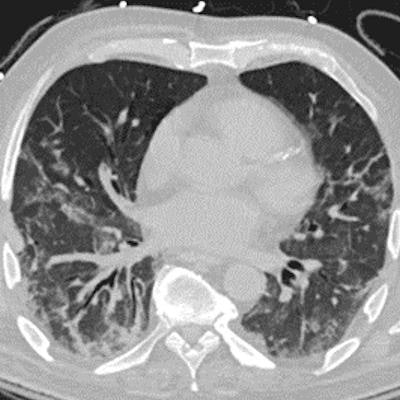
An artificial intelligence (AI) algorithm was able to provide highly accurate predictions of a COVID-19 patient's disease progression and mortality based on analysis of chest CT exams in research published online April 29 in Scientific Reports.
A research team led by first author Janne Näppi, PhD, and senior author Hiroyuki Yoshida, PhD, of Massachusetts General Hospital developed U-survival, a prediction model that combines deep learning-based assessment of chest CT images with a traditional survival analysis statistical model. In testing, U-survival yielded better results than other current prognostication methods.
"Such a model could be used for logistic planning, clinical decision-making, and management of COVID-19 patients," the authors wrote.
The group created U-survival by integrating image information extracted by a pretrained 2D U-Net deep-learning algorithm into an elastic-net Cox proportional hazards model for prognostic predictions. With this approach, a deep radiomic signature for survival analysis is constructed for survival analysis based on the large number of features U-Net has extracted from the images, according to the researchers.
"Thus, we use deep learning as an integral part of the survival model, rather than as a segmentation tool," they wrote.
As it's based on Cox regression analysis, U-survival can provide continuous image-based prognostic predictions, enabling survival probability predictions at any point of time and estimation of survival curves for individual patients. That type of analysis is not available with other prediction models based on logistic regression analysis, according to Näppi and colleagues.
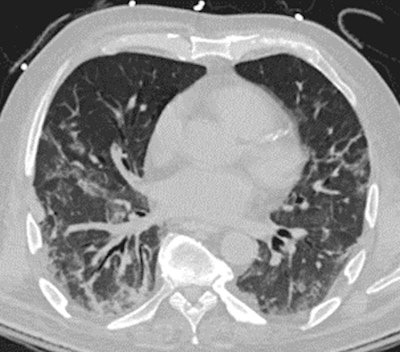
On a dataset of 383 COVID-19 patients from two hospitals, U-survival outperformed laboratory tests (a combination of lactic dehydrogenase, lymphocyte, and C-reactive protein results), as well as image-based visual and quantitative scoring methods such as 4D-curvature of the images, total severity score, and percentage of well-aerated lung parenchyma for predicting disease progression and mortality.
Performance of U-survival AI algorithm for predicting COVID-19 progression and mortality | |||||
Percentage of well-aerated lung parenchyma | Total severity score | 4D-curvature of the CT images | Lab tests | U-survival | |
Progression (concordance index) | 59% | 61.8% | 66.6% | 69.8% | 90.9%* |
Mortality (concordance index) | 53% | 57% | 65.5% | 62.5% | 87.1%* |
In other findings, U-survival produced the largest separation between the Kaplan-Meier survival curves of patients stratified into low- and high-risk groups. This indicates that U-survival was more effective than other methods for stratifying mortality risk in COVID-19 patients, according to the researchers.
"Thus, the U-survival model can be used for providing an automated and objective survival analysis with high accuracy for COVID-19 patients," the authors wrote.
In future work, the researchers are considering an external validation study with a large number of prospective cases and human observers from more than two institutions. In addition, they said they may investigate the potential benefit of a 3D U-Net in the U-survival model and ultimately introduce the algorithm into a clinical routine.