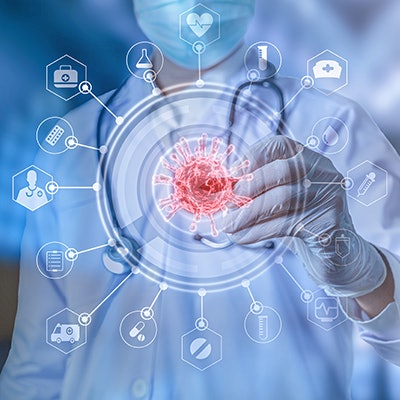
An artificial intelligence (AI) algorithm that utilizes chest CT and laboratory results can be highly accurate for predicting the severity of COVID-19 when patients are being admitted, according to research published February 17 in Infectious Diseases of Poverty.
A team of researchers led by Yibai Xiong and Yan Ma of the China Academy of Chinese Medical Sciences trained three different machine-learning models to identify severe COVID-19 cases at admission based on 23 variables, including chest CT results, one clinical feature, and 21 laboratory values. In testing, the best-performing algorithm yielded an area under the curve (AUC) of 0.970.
This type of prediction model could potentially assist clinicians in promptly identifying patients with severe cases of COVID-19, enabling timely treatment and optimization of healthcare resources, according to the authors.
In their study, the researchers sought to evaluate different machine-learning techniques for the task of predicting COVID-19 severity at admission. They also wanted to determine the most important features utilized by the algorithms in arriving at their findings.
To accomplish these goals, the researchers retrospectively gathered data from 287 COVID-19 patients from JinYanTan Hospital between January 26 and March 28, 2020. For the purposes of the study, they categorized COVID-19 cases as either severe or nonsevere; mild and moderate cases were classified as nonsevere, while severe and critical cases were considered to be severe.
The authors first performed tenfold cross-validation to determine the best demographic, clinical, laboratory, and imaging features to utilize during algorithm training. From this process, they winnowed down 86 features to 23: one clinical, one imaging (either no lesions, unilateral lesion, or bilateral lesions found on chest CT), and 21 laboratory features.
After partitioning the training data into 10 folds, or sets, the researchers trained and tested three different types of machine-learning algorithms -- random forest, support-vector machine, and logistic regression -- 10 times. For each iteration, one of the folds served as the test set. The random-forest machine-learning model was the winner, as determined by average performance for the 10 testing rounds.
Performance of machine-learning model for predicting COVID-19 severity | |
Random-forest machine-learning model | |
Sensitivity | 96.7% |
Specificity | 69.5% |
Accuracy | 84.5% |
Area under the curve | 0.970 |
Delving further into the results, the researchers found that the top 10 features contributing the most to the model's severity predictions were the following:
- Chest CT features
- Neutrophil-to-lymphocyte ratio
- Lactate dehydrogenase
- D-dimer
- Ca2+
- Erythrocyte sedimentation rate
- Alpha-fucosidase (AFU)
- Fever
- Red cell volume distribution width
- Hemoglobin concentration (HB)
The high importance of chest CT findings for the model in predicting severe COVID-19 cases "can help clinicians a fast diagnosis and severity assessment of COVID-19 even before molecular test results are available or laboratory testing is limited," the authors wrote.
Among the important lab results, the researchers highlighted the inclusion of AFU and HGB -- two findings that have been rarely cited in prior studies involving severe COVID-19.
"We also found that AFU is a significant lab indicator to distinguish severe illness, which may be a possible therapeutic target in the future," they wrote.
Other studies in the literature have reported that COVID-19 patients had lower HB and red blood cell count but did not find significant differences between those who survived and those who didn't, according to Xiong, Ma, colleagues, and the National Traditional Chinese Medicine Medical Team.
"Our study indicates that [the random-forest machine-learning model] may be a prioritization tool in predicting COVID-19 severity with favorable overall performance at admission," the authors wrote.