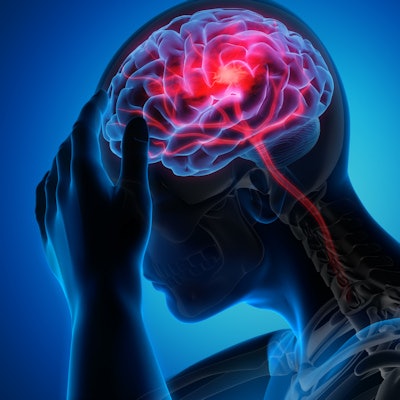
Artificial intelligence (AI) was able to detect large vessel occlusions (LVO) on CT angiography (CTA) and predict treatment outcomes in research published September 15 in the Journal of Stroke and Cerebrovascular Diseases.
In a preliminary study, a multinational research team led by Shujuan Meng and Thi My Linh Tran of Central South University in Changsha, China, trained two 3D convolutional neural networks, one to detect LVO and the other to predict, from CTA and clinical data, the 30-day outcome after mechanical thrombectomy. Their platform produced an area under the curve (AUC) of 0.74 for detection of LVO and 0.82 for predicting patient outcome on a test set of cases.
"The system will be helpful with the management of the LVO stroke care and improvement of patient outcomes," the authors wrote.
LVOs are the cause of about one-third of all acute ischemic strokes. However, they also lead to 90% of the mortality and disability in these patients, according to the researchers.
"To reduce futile recanalization and improve the management of LVO patients, rapid detection of LVO is essential given the potentially rapid decrease of salvageable tissue and the limited treatment window for [mechanical thrombectomy]," the authors wrote. "Furthermore, LVO prediction of clinical outcome for patients who undergo [mechanical thrombectomy] will improve personalized stroke care, as it could help assisting doctors with treatment decisions making as well as identification of further treatment targets (e.g., adjuvant treatment with neuroprotectants, etc)."
They trained and tested their two CNNs using the thin-axial series of 8,650 CTA exams performed at Rhode Island Hospital between 2015 to 2019. Of these cases, 1,021 had an LVO while 7,629 were normal.
The LVO detection model was trained using CTA images from all 8,650 patients and then tested using cross-validation. The outcome prediction algorithm was trained and tested from a dataset of 323 patients with LVOs that were treated with mechanical thrombectomy. Of the 323 patients, 80% were used for training and 20% were set aside for testing.
Performance of AI platform for detecting LVO and predicting treatment outcome | ||
LVO detection model | Outcome prediction model | |
Sensitivity | 61% | 89% |
Specificity | 74% | 66% |
AUC | 0.74 | 0.82 |
The researchers noted that the detection model's sensitivity and specificity need to be considered in the context of the clinical application. With a suspected LVO case, it's far more important to have a lower false-negative rate than to focus on false positives.
"While it can be cumbersome to receive false alarms, a false-positive carries less risk to the patient than a false negative," the authors wrote.
As a result, future iterative detection models should focus on improving the specificity to limit the false-negative cases.
"In practice, every CTA for potential ischemic stroke must be evaluated by the radiologists, and this pipeline only serves as a support tool to expedite triaging, transporting, and treatment," the authors wrote.
The researchers have performed preliminary, behind-the-scenes testing of the AI platform integrated into the network of Rhode Island Hospital. CTA data will be collected in real-time and processed by the model. If an LVO is detected, interventionists will be notified, according to the firm. Radiologists will also be alerted.
"This pipeline can be especially useful at peripheral and smaller centers that lack experienced staff and resources for rapid scan interpretations," they wrote.
The researchers have made their code available on GitHub.