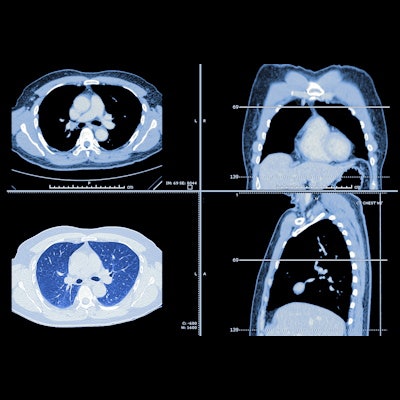
Deep-learning algorithms could be used to determine whether an individual could safely undergo low-dose CT (LDCT) lung cancer screening at biennial intervals rather than annually, suggest findings published March 16 in JAMA Network Open.
The findings could allow clinicians to better tailor lung cancer screening intervals for their patients, a team led by Rebecca Landy, PhD, from the National Cancer Institute in Bethesda, MD, wrote. Landy's group found that a deep learning algorithm helped predict not only an individual's one-year lung cancer risk but also to estimate risk of a one-year screening delay.
"Deep learning algorithms could prioritize people for workup of suspicious nodules and decrease screening intensity for people with low-risk nodules, which may be vital for implementation in healthcare systems," Landy and colleagues wrote.
The National Lung Screening Trial (NLST) showed that annual LDCT screening of high-risk individuals who ever smoked reduces lung cancer mortality. With annual screening though, the chance of finding a lung nodule without a verified cancer by the one-year follow up can be high.
Biennial screening has been explored as a safe, cost-reducing method for low-risk individuals, and deep learning algorithms applied to CT images of lung nodules have shown improved accuracy in classifying malignancies. But assessments of how these algorithms perform for predicting risk of future disease have been lacking.
The Landy team sought to identify low-risk individuals in the NLST and estimate how many lung cancers would have been delayed one year in diagnosis had people been assigned to biennial screening. The group used three methods to attempt to predict whether an individual would be a good candidate for biennial lung cancer screening: a recalibrated lung cancer prediction convolutional neural network (LCP-CNN); the lung cancer risk assessment tool (LCRAT + CT), a statistical model combining individual risk factors and LDCT image features; and the American College of Radiology's (ACR) Lung-RADS version 1.1 metric for lung nodules.
The study included 10,831 LDCT images from patients with presumed nonmalignant lung nodules. Of these, 195 were diagnosed with lung cancer at follow-up screening. The researchers found that recalibrated LCP-CNN had the highest performance of the three methods.
Comparison of predicting one-year lung cancer risk | |
Method | Area under the curve |
LCP-CNN | 0.87 |
LCRAT + CT | 0.79 |
Lung-RADS | 0.69 |
Additionally, if two-thirds of screens with nodules were assigned to biennial screening, recalibrated LCP-CNN would show a significantly lower absolute risk of a one-year delay in cancer diagnosis at 0.28%. LCRAT + CT and Lung-RADS had higher risks at 0.6% and 0.97%, respectively.
"To delay only 10% of cancer diagnoses at one year, more people would have been safely assigned biennial screening under LCP-CNN than LCRAT + CT," the team wrote. For LCP-CNN, this was 66.4% compared to 40.3% for LCRAT + CT.
The authors suggested that risk-based approaches may inform lung cancer screening intervals and noted that this could help screening programs to evaluate the cost-effectiveness and feasibility of delivering interventions that make the most of existing healthcare capacity.
"Our findings suggest the potential to individualize the entire lung cancer screening continuum of care by continually updating risks from entry to exit, via integration into electronic health record clinical workflow," they wrote.