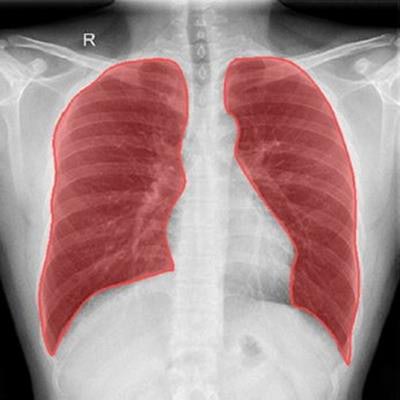
A new two-stage process for using artificial intelligence (AI) to grade the severity of COVID-19 infection on chest x-rays could work better than existing AI-based algorithms, say researchers who described their process in a paper published July 27 in Scientific Reports.
Researchers from the U.S., Italy, and Russia put nine algorithms to the test in a two-stage process, first for lung segmentation and then for disease segmentation and scoring. They found that their best-of-breed combination approach worked better than existing models for COVID-19 analysis of x-ray images.
"Central to our approach is the utilization of two core independent stages which allow us to investigate the regions of interest on an X-ray image," wrote a team led by Viacheslav Danilov, PhD, a senior machine learning engineer at Quantori, a developer of software for the life sciences and biomedicine.
Over two years after the SARS-CoV-2 virus began spreading across the globe, the COVID-19 pandemic remains the world's major health challenge. Being able to screen hospitalized patients quickly to determine the severity of their infection can help direct their care, with x-ray emerging as a preferred tool compared with CT for a variety of reasons, the authors stated.
But determining the extent of COVID-19 infection from chest x-rays is a "nontrivial and time-consuming task," they noted. Tools like AI could help, but so far, the technology has mostly been focused on identifying infection and distinguishing it from other forms of pneumonia.
But what if AI were enlisted to also assess the severity of COVID-19 infection? The researchers decided to test this hypothesis by developing a two-stage process that uses AI to score the extent of disease using image segmentation and multitask learning.
To develop their process, the researchers analyzed nine widely available AI algorithms for lung disease analysis and segmentation to find the best combination. They tested all of the combinations against publicly available datasets that were developed for testing both lung and disease segmentation.
The AI workflow proposed by the group for analyzing is based on two independent stages:
Stage I: Lung segmentation. Nine AI algorithms were employed for various segmentation tasks and trained with data from three publicly available datasets, known as the Darwin, Montgomery, and Shenzhen datasets. Each dataset has different characteristics than can aid in algorithm training -- for example, images in the Darwin dataset include most of the heart, which can demonstrate opacities behind the heart that can assess viral pneumonia severity.
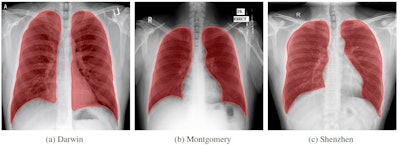
The algorithms performed various tasks, such as excluding unnecessary areas, extracting lung data, and cropping and resizing to exclude areas outside the lungs. Of the nine algorithms, the group found that the DeepLabV3 + model performed best.
Stage II: Disease segmentation and scoring. In this phase, the researchers trained the algorithms on four publicly available COVID-19 datasets: Actualmed COVID-19 Chest X-ray Dataset (ACCD), COVID-19 Radiography Database (CRD), COVID Chest X-Ray Dataset (CCXD), and COVID Chest X-ray Dataset (FCXD). These datasets were compiled with COVID-19 images from 40 different medical institutions.
Stage II involves a multitask learning model with branches that perform classification and segmentation of the data. This model outputs the probability of an image being normal or being a case with disease, the authors noted. For segmentation and scoring, the group found the best-performing algorithms to be based on U-net, FPN, or MA-net.
Next, the authors assessed the performance of the two-stage process against ground truth, which was determined by two senior radiologists who annotated radiographs and graded their severity.
Which algorithms performed best in the two-stage process? In particular, the researchers preferred using the DeepLabV3 + model for lung segmentation, and the MA-Net algorithm for disease classification and segmentation. This combination achieved the lowest mean absolute error (MAE -- lower is better).
Meanwhile, the researchers found that two scoring models that are considered state of the art -- BS-net and COVID-Net-S -- were considered "not suitable" for use on chest-ray exams of healthy patients. Their error rates were high enough that they could lead to the classification of healthy patients as having mild or intermediate COVID-19.
In the end, the researchers said their two-stage process for analyzing COVID severity on chest x-ray could be more useful than existing publicly available algorithms.
"Having compared the solution with the state-of-the-art BS-net and COVID-Net-S, we found our proposal to be more stable in terms of accuracy and more time-efficient in terms of prediction speed," the authors concluded.