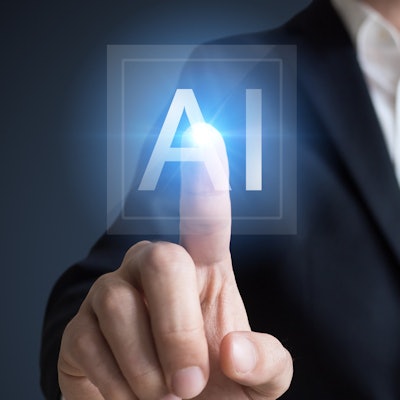
An artificial intelligence (AI) algorithm was able to classify bone lesions with a very high level of sensitivity, potentially enabling rare but often deadly bone tumors to be detected sooner, according to research from Israel.
In testing, a deep-learning model developed by a team from Rambam Health Care Campus achieved over 96% sensitivity and an area under the curve (AUC) of 0.941 for identifying radiographs containing bone lesions.
"We were able to create an AI system to classify radiographs for bone lesions with low false negatives," said Dr. Bar Rinott, who presented the research team's results at ECR 2022 in Vienna.
Primary bone tumors are rare, making up about 4%-7% of all malignant disease in children and adolescents in the U.S. Osteosarcoma and Ewing's sarcoma are the most common bone cancers. Despite their rarity, bone tumors are the third-leading cause of death in young patients aged 0-20 with malignant disease, according to Rinott.
Radiography is frequently the initial imaging study used for evaluation. In bone tumors, clinical findings are often not specific and it's difficult to identify these tumors on x-rays, especially when the disease is at an early stage, he said. This can result in delayed diagnosis and treatment.
As a result, the Rambam group sought to develop a deep learning-based classifier for
bone lesions. They first retrospectively assembled a dataset of 10,000 normal and abnormal radiographs from 1,300 patients. Of these, 8,531 radiographs were excluded because they were either not relevant to the diagnosis, were low quality, or were postoperative studies.
The remaining cohort of 1,177 limb radiographs from 310 patients included 630 normal limb radiographs from 181 patients and 547 abnormal limb radiographs from 129 patients. These images were used to train a deep neural network to perform binary classification of exams as either normal or abnormal.
The goal of the effort was to develop an algorithm that would produce as few false-negative results as possible for bone lesions, according to Rinott.
The researchers used approximately 74% of the dataset for training, about 13% for validation, and around 13% for testing. The researchers then trained, validated, and tested a MobileNet deep-learning algorithm that had been pretrained on the ImageNet database.
AI algorithm's performance on test set for identifying bone lesions on x-ray exams | |
Sensitivity | 96.6% |
Specificity | 82.2% |
Accuracy | 88% |
AUC | 0.941 |
Rinott noted that the algorithm also produces an attention map to show the lesion locations on the radiographs and to help users to understand the AI system's results.
In clinical use, the algorithm could potentially be deployed to alert radiologists that the radiograph could contain a bone lesion, he said.
He acknowledged the limitations of the study, including its reliance on a single site, a single image database, and on patients with an age range of only 5 to 40.