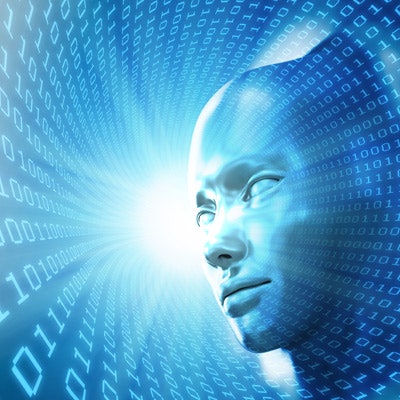
Artificial intelligence (AI) algorithms can identify a patient's demographic information with a high level of accuracy on chest x-rays, according to research published August 11 in the Journal of the American College of Radiology.
After training convolutional neural networks using over 55,000 chest x-rays, a multi-institutional team of researchers led by Dr. Jason Adleberg from Icahn School of Medicine in New York City found that an AI algorithm was nearly perfect for predicting a patient's self-reported gender. Other models also yielded high performance for identifying age groups and ethnicity.
Many deep-learning algorithms are trained using large, public medical image databases. However, these databases may not fit the diversity of patients the model would be used for, according to the group.
In an attempt to explore how well deep-learning algorithms could classify demographic information, the researchers trained four models using 55,174 posteroanterior (PA) radiographs in the MIMIC-CXR database. The algorithms were each trained to perform a specific task: predicting a patient's self-reported gender, self-reported ethnicity, age group, or insurance status.
The study authors then performed two external validations on the models using 29,452 radiographs from 20,573 patients in Stanford University's CheXpert database and another database from a multihospital urban healthcare system in New York City that included 400 radiographs for age groups, 200 for evaluation of self-reported ethnicities, 150 for insurance status, and 100 for self-reported gender.
The model for self-reported gender performed best while the algorithms for age groups and self-reported ethnicity also were highly accurate. However, the algorithm for predicting a patient's insurance status only performed moderately, as measured by area under the curve (AUC).
Performance of AI algorithms for predicting patient demographics from chest x-rays | ||
CheXpert database | Urban hospital dataset | |
Self-reported gender | 0.994 | 0.997 |
Age groups | 0.854 | 0.892 |
Self-reported ethnicity | n/a | 0.832 |
Patient insurance status | n/a | 0.675 |
"These models could be used for quality control on a large database of chest x-rays to ensure that various demographics are equally represented in the development of future deep learning models," the authors wrote. "This technology could also be used to assist in the identification of unidentified patients, particularly with age."
Furthermore, the technology could also be used to fill in missing demographic data on large numbers of discharged patients, according to the authors.
Adleberg and colleagues also emphasized the importance of visualization techniques such as heat maps for ensuring that deep-learning models function as they were intended and to demonstrate anatomical regions of interest for different ethnicities.
"The model highlighted bilateral lung apices in patients who self-identify as African American, and the cervical trachea in patients who self-identify as Asian," they wrote. "Further research could confirm cardiothoracic anatomic variation in different demographic populations."
Deep-learning models may learn unintended causes related to race and incorporate them into decision-making, the authors noted.
"As such, AI software could think a study has a positive or negative finding based on the confounding variable of race," they wrote. "Medical professionals that utilize AI algorithms need to be aware of the potential for bias in the creation of these algorithms. Moreover, the regulatory guidance for machine learning software, which is still in its infancy, does not currently evaluate machine learning applications for potential sources of bias."
To help, the researchers released a publicly available open-source model to provide predictions of age, sex, ethnicity, and insurance status.
"This open-source software could be used to see exactly how diverse a collection of training data truly is, thereby ensuring generalizability of AI models," they wrote.