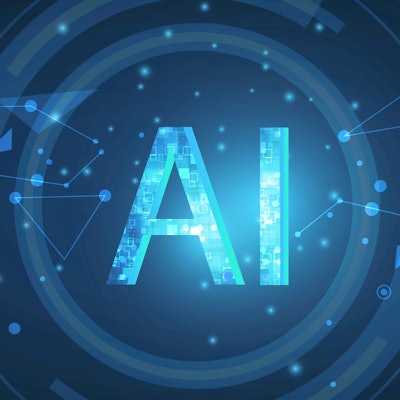
Artificial intelligence (AI) for medical imaging is susceptible to bias based on racial or socioeconomic factors and must be monitored for this, according to a commentary published October 5 in the Journal of the American College of Radiology.
If bias in AI tools for medical imaging isn't identified and removed, patients could be negatively affected, noted authors Dr. Madison Kocher of Duke University in Durham, NC, and Dr. Christoph Lee of the University of Washington in Seattle. The two wrote the commentary in response to a study the Journal of the American College of Radiology published August 11 that found AI algorithms can identify a patient's demographic information with a high level of accuracy on chest x-rays.
"The prospect of sensitive sociodemographic characteristics being identifiable by AI presents a real risk of deployed models using race and other personal characteristics and incorporating them into subsequent medical decisions unbeknownst to radiologists, referring providers, and patients alike," Kocher and Lee warned.
AI algorithms have the potential to help radiologists identify disease and predict patient outcomes, but it's becoming clear that these algorithms may be problematic in that they can also identify the racial and sociodemographic characteristics of patients being imaged. An animated discussion about how to deal with this problem has already begun: In fact, a recent study suggested that proper data handling is crucial for mitigating imaging AI bias.
But what else can radiology do to make sure that AI algorithms don't lead to healthcare bias or worsen existing disparities in patient outcomes based on factors such as race? It's a question of great importance, especially since it's likely that use of AI for medical imaging will continue to increase, Kocher and Lee cautioned.
"It will thus be incumbent on the radiology community to address the real possibility that these AI systems may perpetuate racial disparities and biases in their decision-making abilities without human detection," they wrote.
The two suggested the following to protect against these trends:
- Enlist the support of the American College of Radiology's (ACR) Data Science Institute when training AI algorithms. The institute offers a tool called Certify-AI, which could be used for both external validation of an AI algorithm and ongoing screening for bias.
- Urge government bodies and industry to set standardized population-level registries that could be used to evaluate and monitor medical imaging AI algorithms for bias.
In any case, the effort to monitor imaging AI for bias will be ongoing, according to the team.
"Ensuring integrity of [medical imaging] AI algorithms is not only necessary prior to release, but periodic quality control will be important to assess for acquired biases and AI drift over time," Kocher and Lee concluded.