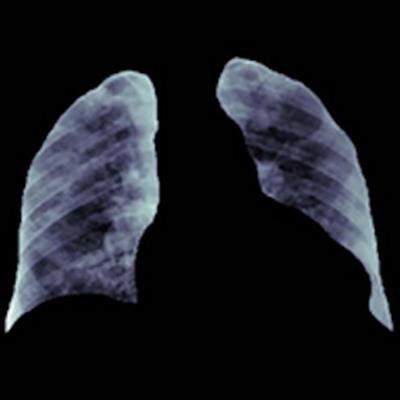
Bias must be minimized in radiology artificial intelligence (AI) algorithms to enable clinical adoption. And that process should begin with proper data handling, according to an article published August 24 in Radiology: Artificial Intelligence.
In the first of a three-part special report on handling bias in radiology AI, researchers led by first author Dr. Pouria Rouzrokh and senior author Dr. Bradley Erickson, PhD, of the Mayo Clinic in Rochester, MN, reviewed 12 suboptimal practices that can occur during handling of data used to train and evaluate radiology AI algorithms. They also shared potential mitigation strategies.
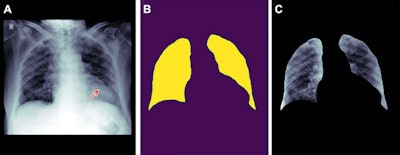
"If these systematic biases are unrecognized or not accurately quantified, suboptimal results will ensue, limiting the application of AI to real-world scenarios," Erickson said in a statement from the RSNA.
Three suboptimal practices can occur for each of the four steps in handling data for training AI algorithms, including the following:
- Data collection: improper identification of the dataset, single source of data, and unreliable source of data
- Data investigation: inadequate exploratory data analysis, exploratory data analysis with no domain expertise, and failing to observe actual data
- Data splitting: leakage between datasets, unrepresentative datasets, and overfitting to hyperparameters
- Feature engineering: improper feature removal, improper feature rescaling, and mismanagement of missing data
"Each of these steps could be prone to systematic or random biases," Erickson said. "It's the responsibility of developers to accurately handle data in challenging scenarios like data sampling, de-identification, annotation, labeling, and managing missing values."
Before training data is collected, a careful planning process should be conducted, including an in-depth review of clinical and technical literature and collaboration with data science experts, according to the authors.
"Multidisciplinary machine learning teams should have members or leaders with both data science and domain (clinical) expertise," he said.
Developers should investigate the data from multiple perspectives to discover properties that may assist in detecting potential data issues, according to the researchers. They offered a number of recommendations for this process, which is known as exploratory data analysis:
- Check the data type heterogeneity.
- Analyze the frequency of the data.
- Perform univariate analysis for nonimaging data.
- Perform multivariate analysis for nonimaging data.
- Explore the timing of studies.
- Evaluate the dimension (aspect ratio) of imaging data.
- Analyze the pixel (or voxel) values.
- Observe the actual imaging data.
More-heterogeneous training datasets can be assembled by collecting data from multiple institutions from different geographical locations and using data from different vendors and from different times, according to the researchers. Public datasets can also be included.
"Creating a robust machine-learning system requires researchers to do detective work and look for ways in which the data may be fooling you," Erickson said. "Before you put data into the training module, you must analyze it to ensure it's reflective of your target population. AI won't do it for you."
The researchers discuss model development in part two of their report on minimizing bias in radiology AI. The last article covers performance metrics.