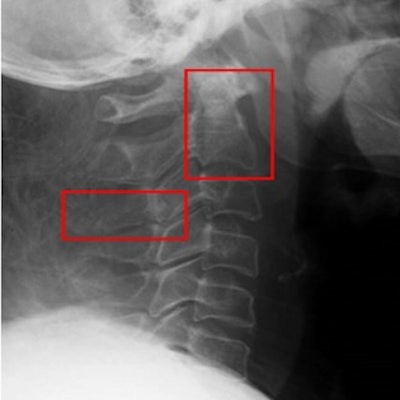
Researchers from Thailand have developed an artificial intelligence (AI) algorithm that may be able to help trauma clinicians diagnose spinal cord injuries on x-rays, according to a study published August 24 in Heliyon.
Researchers at Khon Kaen University's AI and Informatics in Medical Imaging Research Group tested a deep-learning model trained to identify damage on lateral neck or lateral cervical spine (C-spine) x-rays. They then compared its diagnostic performance to that of readers with varying levels of clinical experience.
"Deep learning can enhance the use of lateral C-spine or neck radiography with an accuracy of 75% which may be as high as the detection accuracy of [an] expert's diagnosis," wrote first author Dr. Arunnit Boonrod and colleagues.
Traumatic spinal cord injury (TSI) is a leading cause of morbidity and mortality worldwide, with traffic accidents and falls the most common causes. Cervical spine x-rays alone are insufficient to completely examine these injuries, thus requiring supplemental CT scans, according to the authors.
However, given that cervical spine CT scans are time-consuming, comparatively costly, and not always available in general care, lateral x-rays remain the most widely used screening method to help physicians triage these patients, the authors wrote.
Thus, in this study, the group developed a deep-learning algorithm designed to detect spinal cord damage on x-rays, and the team aimed to assess whether the model could improve the diagnosis of injuries.
The model is based on a machine learning concept for object detection called "you only look once" (YOLO), first developed in 2015 as an alternative to classification-based algorithms like convolutional neural networks (CNN). YOLO models are regression-based algorithms that can be faster than CNNs.
In the current study, the researchers used three versions of YOLO algorithms, applying them to 4,500 images to detect and classify cervical spine injuries. To test the models, they culled 229 x-rays (120 negative and 100 positive) from patients at their hospital who also underwent CT scans, which served as the ground truth. Twenty radiographs of each class were randomly selected for inclusion in the test set.
The x-ray images were also evaluated by a radiologist with nine years of experience, an orthopedist with seven, and a senior radiology resident.
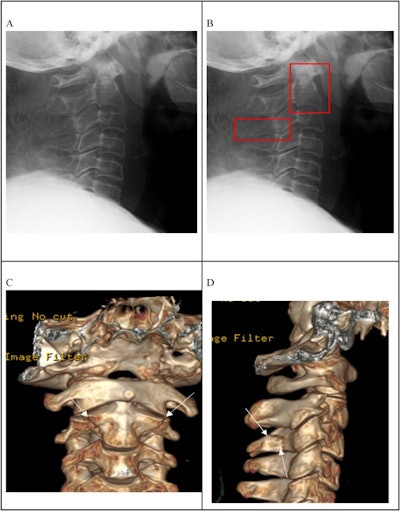
According to the findings, the YOLO-V4 model achieved the highest sensitivity of the three models, at 80%, as well as a specificity of 72% and an accuracy of 80% for detecting spine injury. Compared with the radiology resident, the model had a higher accuracy (p = 0.012) and specificity (p = 0.011), but there was not a statistically significant difference between the model and the radiologist or the orthopedist, the authors wrote.
Predictive values for YOLO-V4 algorithm for diagnosis of C-spine injury | ||||
Resident | Orthopedist | Radiologist | YOLO-V4 | |
Accuracy | 50% | 72.5% | 82.5% | 75% |
Sensitivity | 50% | 65% | 75% | 80% |
Specificity | 50% | 80% | 90% | 72% |
"The model provides a rapid interpretation of plain radiograph results, which can help clinicians evaluate patients with suspected cervical spine injury," the researchers wrote.
Ultimately, aside from helping clinicians in trauma settings diagnose spinal cord injuries on x-rays, an aim of implementing the YOLO-V4 model would be to reduce the need for CT scans, the authors wrote.
Plain radiographs require highly experienced eyes to make an accurate diagnosis, yet an expert's immediate opinion is not always available in traumatic spinal cord injury settings. Subsequent supplemental CT scans can also be negative, which renders them as excessive, they wrote.
"Deep learning can improve the accuracy of lateral C-spine or neck radiographs. We anticipate that this will assist clinicians in quickly triaging patients and minimize the number of unnecessary CT scans," Boonrod and colleagues concluded.