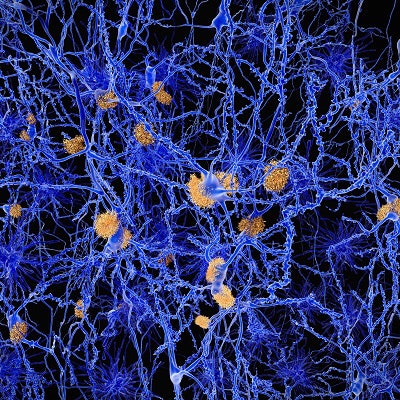
Making use of both gray-matter morphometry and white-matter tractography techniques, the combination of artificial intelligence (AI) and multimodality MRI can accurately predict if a patient will develop Alzheimer's disease, enabling early treatment, according to research published on bioRxiv.org.
A multi-institutional team examined the utility of structural MRI and diffusion MRI as imaging markers of Alzheimer's disease by using machine learning-based analytics and high-throughput brain phenotyping determined from morphometry, as well as whole-brain tractography of the white-matter structural connectome. In testing on image data from a dementia clinic, the researchers found that the machine-learning algorithm could achieve up to 98% accuracy for detecting Alzheimer's disease and 84% accuracy for predicting its precursor -- mild cognitive impairment.
"In sum, this study lends a strong support for white-matter structural connectome estimated using diffusion tractography as a method towards an accurate, clinically useful imaging marker of [Alzheimer's disease]," they wrote.
First author Yun Wang, PhD, and colleagues from Columbia University Medical Center, Brookhaven National Laboratory, Stony Brook University, and Ilsan Hospital in South Korea trained machine-learning models based on structural and diffusion MR images acquired from more than 200 elderly patients who visited a dementia clinic at Ilsan Hospital. The patients had been diagnosed by neurologists as having Alzheimer's disease, mild cognitive impairment, or subjective cognitive decline -- considered to be the earliest sign of dementia (bioRxiv.org, January 30, 2018).
First, several existing algorithms were used to process and analyze the raw MR images. The researchers then applied machine learning to train classification models based on the brain phenotypes resulting from the estimations of morphometric and tractography data in patients from each diagnostic category. The models were then used to predict diagnoses.
The researchers found that the model trained with both morphometric and connectome data more accurately classified Alzheimer's disease and mild cognitive impairment than a model trained with only morphometric data. What's more, the connectome model classified mild cognitive impairment and subjective cognitive decline as accurately as the combined model; the morphometry model did not accurately classify those two conditions.
"The accuracy of our machine-learning models trained on brain connectome estimates surpassed that of existing imaging-based markers used in clinical settings (e.g., white-matter hyperintensities) by 10% and 29%, respectively," said co-author Jiook Cha, PhD, of Columbia University Medical Center in a September 17 statement from Brookhaven National Laboratory. "Using independent data from the Alzheimer's Disease Neuroimaging Initiative, we replicated these results."
Given their findings, the researchers predicted that the structural connectome may contain salient information -- additional to morphometry -- for predicting a subtle clinical outcome instead of just making a current diagnosis. Future research should examine this possibility in retrospective clinical data, according to the researchers.
"Positive results from such a retrospective study will then lead to prospective clinical studies to test clinical utility of connectome-based MRI markers," they wrote.