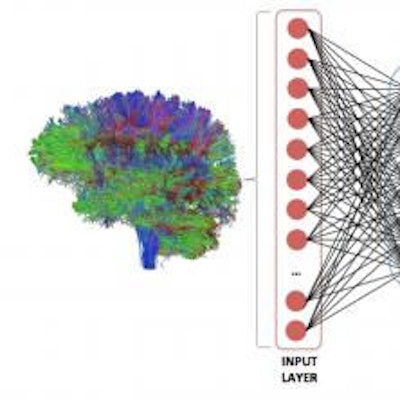
An artificial intelligence (AI) algorithm that analyzes whole-brain structural connectome maps produced from diffusion MRI studies can accurately predict surgical outcomes in patients with mesial temporal lobe epilepsy (TLE), according to research published in the September issue of Epilepsia.
A group led by Dr. Ezequiel Gleichgerrcht and Dr. Leonardo Bonilha of the Medical University of South Carolina (MUSC) trained a deep-learning model that yielded nearly 90% positive predictive value and almost 80% negative predictive value for determining whether a patient would have persistent, disabling seizures or would be seizure-free one year after receiving epilepsy surgery. In comparison, a classification model based on clinical variables alone was less than 50% accurate.
"Deep learning demonstrated to be a powerful statistical approach capable of isolating abnormal individualized patterns from complex datasets to provide a highly accurate prediction of seizure outcomes after surgery," the authors wrote (Epilepsia, September 2018, Vol. 59:9, pp. 1643-1654).
Epilepsy surgery is often recommended for patients who don't respond to medication. However, many epilepsy patients are hesitant to undergo brain surgery due to the fear of operative risks and the fact that only two-thirds of patients are seizure-free a year later, according to the group. As a result, the MUSC researchers sought to apply deep learning to the challenge of analyzing the whole-brain connectome -- produced via analysis of diffusion MRI -- to see if they could predict surgical outcomes.
First, they retrospectively classified 50 consecutive patients with unilateral TLE as having persistent, disabling seizures or being seizure-free one year after surgery. Next, they reconstructed the patients' structural connectomes from their presurgical whole-brain diffusion tensor imaging studies. These connectomes were then used to train a deep neural network to classify surgical outcome.
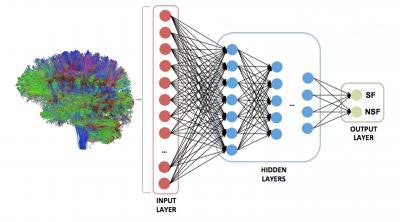
After fivefold cross-validation was performed, the deep-learning algorithm had a mean positive predictive value of 88% ± 7% and mean negative predictive value of 79% ± 8%. A model based on clinical variables alone could only muster 45.5% classification accuracy.
"Using structural connectivity as the input layer, our neural network showed high accuracy to predict seizure outcomes after epilepsy surgery," Gleichgerrcht and colleagues wrote.
The researchers credited features located in the ipsilateral temporal and extratemporal regions and in the contralateral hemisphere for the neural network's high classification accuracy.
As the project was retrospective in nature, the researchers said they would ideally like to perform a multisite prospective study that would analyze presurgical diffusion MRI scans and then follow-up with the patients for at least one year after surgery. The MUSC neurologists also believe that integrating the brain's functional connectome could enhance the algorithm's ability to predict surgical outcomes.
The MUSC initiative "is not a question of human versus machine, as is often the fear when we hear about artificial intelligence," Gleichgerrcht said in a statement from MUSC. "In this case, we are using artificial intelligence as an extra tool to eventually make better informed decisions regarding a surgical intervention that holds the hope for a cure of epilepsy in a large number of patients."