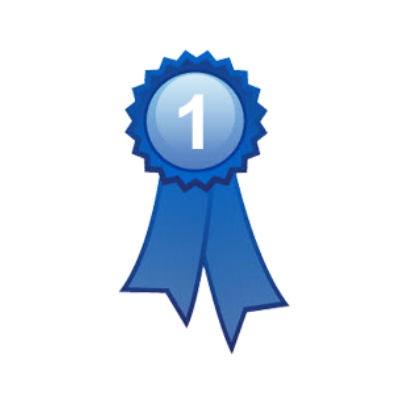
Patients find MRI scans to be uncomfortable; aside from the claustrophobia that wide-bore scanners can induce, patients experience a loud environment and complain of a loss of control, according to presenter Dr. Lawrence Tanenbaum of imaging services provider RadNet. Up to 30% report anxiety reactions.
"Our internal RadNet data suggest that even a 10% reduction in the overall scan time yields significantly higher patient ratings," he said.
Truncated protocols for MRI have been the subject of many publications and presentations over the past few years. Results suggest that while clinical value is preserved, most radiologists prefer to maintain the style and integrity of traditional approaches, Tanenbaum said. In their study, the researchers evaluated the performance of iQMR (Medic Vision Imaging Solutions), a machine learning-based iterative reconstruction algorithm that enables MR scans to be performed with a lower cumulative signal.
"Since building signal requires time, a lower signal requirement means the scans don't have to be as long," Tanenbaum told AuntMinnie.com. "Faster scans are less likely to have motion artifacts as well."
The software reduced MRI scanning time by approximately 30% while producing similar overall image quality, the group found.
Stop by this talk on Tuesday afternoon to learn more.
This paper received a Roadie 2018 award for the most popular abstract by page views in this Road to RSNA section.