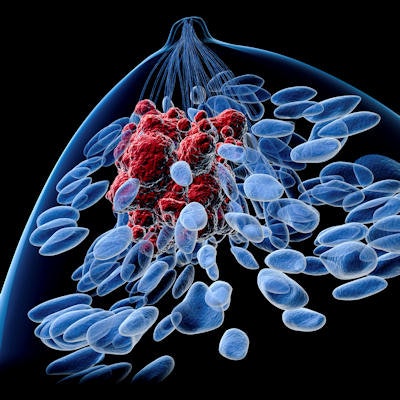
Can an artificial intelligence (AI) algorithm identify the molecular subtype of breast cancer from breast MRI as accurately as genetic testing? Not yet, but a deep-learning model offers considerable potential, according to research published online January 31 in the Journal of Digital Imaging.
In a feasibility study, a research team led by Dr. Richard Ha of Columbia University Medical Center in New York City trained a convolutional neural network (CNN) that yielded 70% accuracy for predicting breast cancer subtypes from analysis of preoperative breast MRI exams.
"Personalized breast cancer risk stratification may be possible with a CNN plus MRI data instead of gene expression profiling," the authors wrote.
Tissue sampling is the current gold standard for predicting breast cancer subtype, with immunohistochemistry (IHC) used as surrogate genetic testing, according to the researchers. However, IHC surrogates can have a wide range of agreement with explicit genetic testing for predicting breast cancer subtype.
"Given the wide spectrum of prognosis and indicated treatment strategies based on tumor subtype, a need exists for more accurate diagnosis to aid in an individualized treatment plan," the authors wrote.
Machine learning has shown promising results in breast MRI for evaluating radiogenomics, which is the process of linking radiomics -- quantitative radiology techniques -- to the hidden genotype of a tumor or tissue. However, the researchers noted that the currently available research in the literature relies on human-extracted phenotypic features to guide machine learning and the subsequent prediction of molecular subtypes using semiautomated methods. As a result, they instead sought to utilize a CNN, which can learn to automatically extract the imaging features needed for the task.
In their retrospective study, the researchers first gathered 216 patients with a known diagnosis of breast cancer, a preoperative MRI, and IHC staining pathology data:
- 74 had a luminal A breast tumor (positive for estrogen receptor [ER] and/or progesterone receptor; negative for human epidermal growth factor receptor 2 [HER2])
- 106 had a luminal B breast tumor (ER- and/or PR-positive; HER2-positive)
- 13 had an HER2-enriched type breast tumor (ER- and PR-negative; HER2-positive)
- 23 had a triple-negative breast tumor (ER-negative, PR-negative, and HER2-negative)
They then trained a CNN using postcontrast MR images and ground-truth labels for the molecular breast cancer subtypes that were obtained from the electronic medical record.
On a testing set of 40 patients that was balanced among these four subtypes, the CNN yielded 70% accuracy, 60% sensitivity, and 95.8% specificity. It also produced a class-normalized macro area under the curve (AUC) of 0.853 and a nonnormalized microaggregated AUC of 0.871, reflecting improved discriminating power for the luminal A and luminal B subtypes that were highly represented in the training datasets.
"Future advances with a larger dataset will likely improve the predictive strength of our model, with the potential to rival accuracy of standard genetic testing in determining breast cancer subtype," the authors wrote.