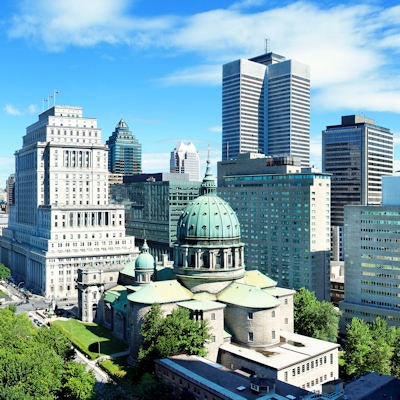
MONTREAL - Artificial intelligence (AI) in medical imaging could open the door for huge advances in healthcare by assisting in diagnosis, the understanding of disease development, and the prediction of patient outcomes, according to a talk at the International Society for Magnetic Resonance in Medicine (ISMRM) annual meeting.
It also could help to speed up and make accurate clinical trials for new treatments, as well as permit advances in personalized medicine, said Tal Arbel, PhD, director of the probabilistic vision group in McGill University's Center for Intelligent Machines.
"We are on the cusp of an industrial revolution in terms of AI and its integration into healthcare," she said. "There's enormous potential for impact in terms of accelerating precision medicine and drug discovery, but [I say that] with caution because there're still challenges that remain."
Clinical use of AI
Although a wide variety of machine-learning methods have been successful for segmentation, classification, and prediction tasks in medical imaging, these methods have yet to be widely integrated into clinical practice, Arbel said. Some of these integration challenges are due to the fact that computer science/engineering labs often don't have access to large-scale annotated datasets required for training, or they don't have access to clinicians or their needs as these methods are developed, she said.
As a result, models are being developed based on smaller, proprietary, or benchmarking datasets. Consequently, these algorithms aren't robust enough to handle the variability in patients and data across centers and scanners, she said. Also, algorithms are being fine-tuned for established metrics that might not be important for the clinical task of interest, she said.
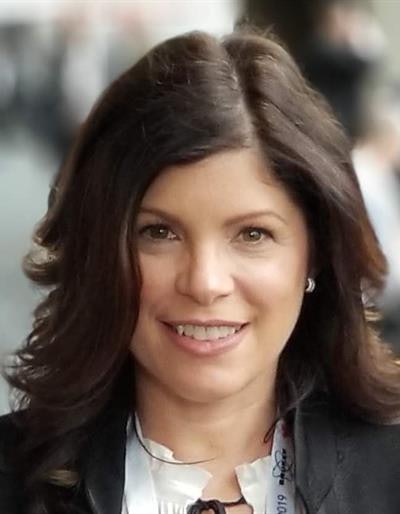
In her talk during the ISMRM's plenary session on Tuesday, Arbel shared her group's work in developing machine-learning techniques for medical image analysis of large clinical trial MRI datasets, specifically for patients with multiple sclerosis (MS). The group has developed algorithms to detect and segment lesions on brain MRI of MS patients, predict future MRI lesion activity at baseline, and predict progression in large datasets of patients with relapsing MS or progressive MS.
"The long-term objective of all of this work is to move towards precision medicine and to help with drug development," she said.
MS is the most common neurological disease affecting young people, and there's no cure. One of the hallmarks of MS is the appearance of brain lesions on MRI. The volume and number of lesions that are hyperintense on T2-weighted images have been used to estimate the burden of disease and activity, according to Arbel.
The appearance of new or enlarging T2 lesions on successive MRI exams is really important as it's a marker for disease activity and needs to be monitored for assessing treatment efficacy, according to Arbel. However, MRI reports are often not sufficiently detailed, accurate, or precise with respect to detection of new lesions, she said. Furthermore, radiologists can find visual identification of new lesions to be challenging.
"So if you subtract the baseline and the follow-up and you look at the difference in image, it's really hard to distinguish new and enlarging lesions from other artifactual changes," she explained.
Gadolinium-enhancing lesions
The detection of gadolinium-enhancing lesions on T1 images is also important as a marker of disease activity. These lesions are also difficult to identify, however, as there's a large amount of nonlesional enhancement on these images, and the lesions are often tiny, she said.
For clinical trials, there needs to be a wide set of robust, accurate measures of MRI lesion endpoints to determine the burden of disease and activity to measure overall treatment efficacy. These measures include the number and volume of T2 lesions, the number of new and enlarging T2 lesions on sequential MRI exams, and the number of gadolinium-enhancing lesions.
Unfortunately, manual or semi-manual delineation of all of the lesions is slow, expensive, and inconsistent, she said.
"So we need an automatic approach, and we need it to be robust against changes across trials, across disease stage, across scanners, and across centers," she noted.
Arbel's group has a long-term collaboration with an industrial partner that provides software analysis to the pharmaceutical industry for MS clinical trials. The team has also received a federal grant to develop a federated progression MS MRI dataset that includes tens of thousands of patient scans from multiple clinical trials at multiple global imaging centers on multiple imaging scanner technologies. The megavoxel MRI volumes in the dataset encompass multiple imaging modalities at multiple time points with expert-generated lesion labels provided by the corporate partner.
For the task of T2 lesion detection and segmentation, the researchers developed a probabilistic machine-learning framework that was trained on images from both 1.5- and 3-tesla scanners. Although their method performed comparably to other techniques overall, it significantly outperformed these techniques for detecting small lesions. That's important because 40% of lesions are small -- ranging from three to 10 voxels, according to Arbel.
Segmenting new, resolving lesions
The researchers also developed a Bayesian machine-learning model to automatically detect and track the evolution of T2 lesions. Their algorithm integrates information from images acquired at different time points and learns probabilistic models for changes in intensity patterns, as well as lesions and/or healthy tissue classes over sequential scans. It's useful for assessing treatment effects in the clinic and in clinical trials, according to Arbel.
In addition, the researchers created a probabilistic machine-learning framework to automatically detect gadolinium-enhancing MS lesions. This model combines voxel interactions and texture features to learn to maximally discriminate between nonlesions and lesional enhancements on the images. It was trained on clinical trial data with 2,380 scans from 247 different centers and then tested on two other clinical trials involving 813 scans from 27 centers and 2,770 scans from 142 centers, respectively.
The machine-learning algorithm for MS lesion detection and segmentation has been placed into the commercial software pipeline of their industrial partner, which has realized improvement in efficiency and precision.
"[That saved] them time and money, but much more importantly, improved treatment analysis," she said. "These software packages are used pretty much for all of the development of almost all of the new MS drugs used worldwide."
Deep learning?
Given the recent success with using deep learning in a variety of medical imaging tasks such as segmentation, the researchers also wanted to explore that technology's potential for MS applications.
To explore whether deep learning could provide added value, the researchers developed a deep-learning framework for detecting and segmenting T2 lesions. In testing on 1,068 MS patient brain MR images from a large, multicenter, and multiscanner clinical trial, the researchers found that the algorithm performed well for large and medium lesions. That wasn't the case for small lesions, however.
"It had very low sensitivity for small lesions," Arbel said.
Clinicians would therefore not trust in the system, leading to reluctance in adopting deep-learning techniques in clinical practice, she explained. The group therefore explored the use of uncertainty estimates, in which the deep-learning framework quantifies the reliability of predictions. Predictions could be categorized into one of three classes: lesion, nonlesion, and uncertain cases.
"The clinician can then review the areas that are more uncertain, and that's something that we've explored," she said. "In this way, uncertainty estimates can be part of the framework where deep learning is therefore used to accelerate the clinical [workflow] and not replace it. Uncertainty [estimates] build trust, and that enables faster review."
The team developed a deep-learning framework to produce different uncertainty measures in detecting and segmenting focal pathologies such as MS lesions.
"What we found is that when our system is correct, it generally has high confidence, but when it's incorrect, it actually has higher uncertainty," Arbel noted.
The researchers also discovered that filtering out uncertain predictions increases the voxel-level and lesion-level performance on the remaining certain predictions, according to Arbel.
"That's good news because then clinicians can trust the most confident results and review the other ones," she said.
Predicting future lesion activity
They also explored the use of deep learning for predicting future lesion activity from baseline MRI.
"This can help us learn a bit more about the natural disease worsening, but also [help with] risk assessment and treatment selection," Arbel added. "If our system tells you that the patient will have a lot of new lesions in a year, potentially you could then decide to give a more aggressive treatment."
The ability to predict future lesion activity would also help to identify potential treatment responders, she said.
The team trained a deep-learning network to predict future new or enlarging T2 lesions or gadolinium-enhancing lesions from baseline MRI and lesion labels. In testing on 937 patients from an international multisite, multiscanner clinical trial dataset, the method yielded 80% sensitivity, 79% specificity, and an area under the curve (AUC) of 0.88.
Predicting disability progression
Currently, the group is working on early prediction of future clinical disability progression for MS. This capability would lead to improved patient care and more appropriate treatment selection, according to Arbel. However, no MRI biomarkers are currently available for reliably predicting future disease progression, she said. In fact, lesions are not well correlated with future disease progression.
As a result, the team developed an end-to-end deep-learning model for predicting MS disease progression within one year of baseline predicated on baseline imaging alone. In testing on 1,083 patient scans from two international clinical trial datasets, the algorithm yielded an AUC of 0.701 for predicting progression. It also provides uncertainty measures for its decision.
A number of challenges are being addressed with deep learning, including understanding how these networks arrive at their conclusions. In Arbel's lab, for example, the group is working to find out -- via attention maps -- why the algorithm is confident in its predictions for MS progression.
"This is important because it would understand disease course better and also help us develop new MRI biomarkers for disease progression," she said.