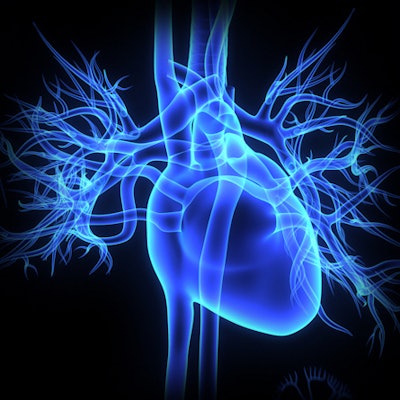
Artificial intelligence (AI) can dramatically reduce the time it takes to analyze heart function on cardiac MRI scans with no significant difference in accuracy compared to human interpreters, according to a study published online September 24 in Circulation: Cardiovascular Imaging.
A data-trained algorithm accurately analyzed cardiac MRI scans of heart function in approximately four seconds, compared with approximately 13 minutes for a team of trained cardiologists.
"Based on multicenter, multivendor, multifield strength, multidisease data, a 9% change in ejection fraction can be detected confidently by expert clinicians, and this is similar using automated analysis," wrote the group led by Dr. Charlotte Manisty, PhD, from University College London. "Given that a major source of measurement variability is attributable to the observer, automated approaches offer the future potential to surpass human experts, demonstrable using this scan-rescan resource."
MRI is the modality of choice to evaluate left ventricular structure and function, including ejection fraction, which is key for assessing patients with cardiovascular disease. However, even when cardiovascular MRI is performed at high resolution, analyses can vary considerably between readers.
One potential solution is to use machine-learning techniques and deep-learning neural networks to reduce intra- and interobserver variability. First, however, the technology must prove that it can perform better than humans.
To help determine machine learning's worth, the researchers collected cardiac MR images from 599 cases at five U.K. facilities that housed six different 1.5- and 3-tesla MRI scanners (Avanto and Aera, Siemens Healthineers; Achieva, Philips Healthcare). They then created a final dataset of 110 scan-rescans from patients with myocardial infarction, left ventricular hypertrophy, cardiomyopathy, and other pathologies, as well as a group of healthy subjects, in order to train a neural network to interpret the results.
"Precision [of the machine-learning algorithm] can only be assessed using a test:retest dataset -- this requires a significant sized patient cohort to be scanned twice in an identical fashion within an interval short enough to effectively exclude variation in disease biology," the authors explained. To that end, MRI scans and rescans were performed within one week, 87 of which (82%) were completed on the same day.
One cardiologist with more than 15 years of experience and two cardiology trainees with less than one year of experience provided the human interpretations.
The researchers found good agreement between the expert, the two trainees, and the machine-learning algorithm for all left ventricular metrics, including left ventricular ejection fraction (LVEF), for which there was no statistically significant difference in variation between the trio and the machine-learning approach.
Variation in performance for left ventricular ejection fraction | |||
Expert | Trainees | Machine learning | |
Variation | 6.1% | 8.3% | 8.8% |
p-value | 0.258 | 0.365 | 0.862 |
Considering the reduction in interpretation time from 13 minutes for the expert and trainees to four seconds with machine learning, the researchers extrapolated a savings of 54 clinician days per year, based on more than 150,000 cardiac MRI scans performed annually in the U.K.
"We believe that, with improvement, it is only a matter of time before automated approaches are superhuman," they concluded.