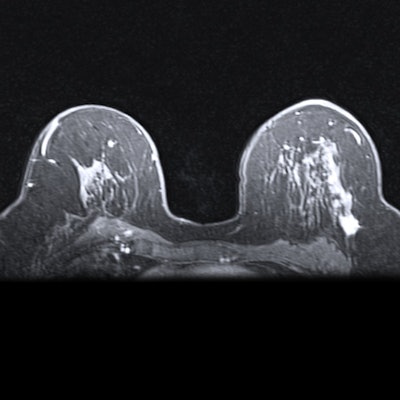
Using a deep-learning computer-aided diagnosis (CADx) algorithm with multiparametric MRI (mpMRI) improves radiologist performance in identifying breast cancer, according to a study published June 29 in Nature.
The combination of these technologies could translate to better patient care, wrote a team led by PhD candidate Qiyuan Hu of the University of Chicago.
"[Our] proposed deep transfer learning CADx method for mpMRI may improve diagnostic performance by reducing the false-positive rate and improving the positive predictive value in breast imaging interpretation," the group wrote.
MRI has become a valuable tool for detecting and characterizing breast cancer, and dynamic contrast-enhanced (DCE) MRI offers further benefit of morphological and functional lesion information. But DCE-MRI has moderate specificity, which can lead to unnecessary workups and biopsies, the group noted.
To address this problem, researchers have been exploring the use of other MRI sequences, such as T2-weighted MRI with DCE-MRI data, an approach called multiparametric MRI. And adding artificial intelligence to mpMRI could pack even more clinical punch, according to the researchers.
Hu's group investigated the performance of three CADx methods that incorporate AI, combining information DCE-MRI offers with T2-weighted MRI protocols.
The three methods included the following:
- Classifier fusion: Averaging DCE and T2-weighted outputs to estimate malignancy
- Feature fusion: Using convolutional neural network (CNN) features taken from both DCE and T2-weighted MRI as classifier input
- Image fusion: Fusing DCE and T2-weighted imaging into one image
The researchers then compared these three methods with the two single-sequence methods, DCE-MRI and T2-weighted MRI.
The study included 927 MR images of lesions (21% benign, 79% malignant) taken from 616 women. Each exam consisted of a DCE-MRI sequence and a T2-weighted sequence; the researchers used a CNN to extract features from these two sequences and train the deep-learning algorithm to distinguish between benign and malignant lesions. They evaluated the algorithm's performance using the area under the receiver operating curve (AUC) measures.
The feature-fusion method outperformed DCE-MRI alone, while the classifier and image fusion methods did not show statistically significant improved performance. However, all three mpMRI approaches outperformed T2-weighted MRI alone.
Performance of CADx with AI and various MRI sequences for breast cancer | |||||
Performance measure | DCE | T2-weigtted | Classifier fusion | Feature fusion | Image fusion |
AUC | 0.85 | 0.78 | 0.86 | 0.87 | 0.85 |
Sensitivity | 75.9% | 69.8% | 77.6% | 77.9% | 76.5% |
Specificity | 76.5% | 72.7% | 77.1% | 78.5% | 77.1% |
Positive predictive value | 89.7% | 87.3% | 90.1% | 90.7% | 90% |
Negative predictive value | 54.2% | 47.3% | 56.2% | 56.9% | 55% |
The study results should help guide the use of MRI in breast cancer detection and suggest further research directions, according to the group.
"Our study proposed a mpMRI approach that significantly outperformed the CADx benchmark that uses DCE alone in the task of distinguishing between benign and malignant breast lesions," the group concluded. "Future work will expand the analysis to include other valuable MRI sequences, such as diffusion-weighted imaging."