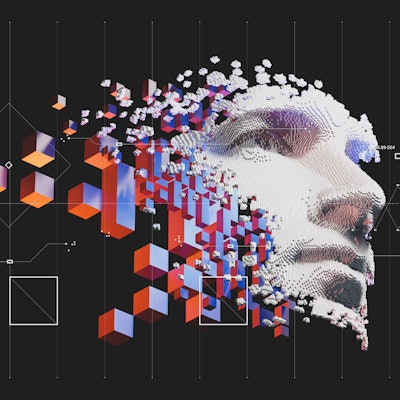
Artificial intelligence (AI) shows potential to have a substantial clinical impact in a wide range of applications in MRI, but image interpretation isn't on the immediate radar, according to an August 8 presentation at the Society for MR Radiographers & Technologists (SMRT) meeting.
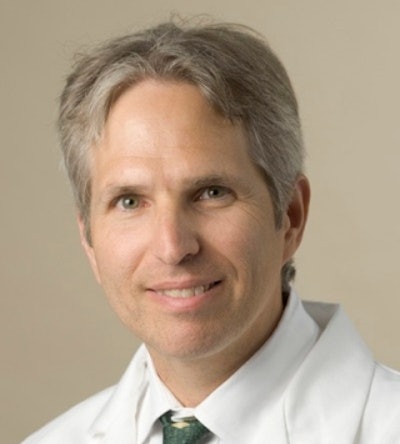
Instead, AI-based image reconstruction and postprocessing methods are likely to be implemented first, said Dr. Greg Zaharchuk, PhD, of Stanford University in Palo Alto, CA.
"Whether these will be killer apps will depend on whether sites really want to be more efficient," he said. "This may be driven by U.S. healthcare mandates and reimbursement changes over time."
Image interpretation will probably be the last category of potential AI applications for MRI to be implemented, but tools that assist the radiologist might find some traction, Zaharchuk said. Other new applications such as using AI to enable lower or even no doses of gadolinium-based contrast are also emerging as "real wild cards, but could revolutionize how we do imaging," he said.
Killer apps
To be a killer app in radiology, AI has to address an important clinical need, Zaharchuk said. Furthermore, the current solutions must be suboptimal and the method must be easy to implement and robust. Also, it needs to have commercial support in order to be used.
In AI, some not-so-great applications include those that focus on rare diseases or tasks that may be very easy for radiologists to do already. They may address topics that don't impact patient care, or are very sensitive to the type of inputs, such as scanners or populations. Or there may be a lack of ground truth in the data used to train the algorithms.
Zaharchuk said that using AI to diagnose COVID-19 on CT scans is an example of a not-so-great radiology application. This is a task that is relatively easy to do for radiologists, and it also doesn't really impact patient care, he said.
"You still need to test the patients, and we know that patients can be asymptomatic and have normal studies as well," he said.
A good AI application
A good AI application should address common but tedious clinical tasks, Zaharchuk said. In addition, humans would pretty much agree all of the time on the desired results from the algorithm. The app also needs to push the boundaries of what might be possible with time or contrast, be robust to minor imperfections, and be easy for the radiologist to overrule, he said.
An example of a good AI application could include, for example, finding and segmenting brain metastases -- an important task for neuroradiologists.
"Each one of these lesions can be targeted separately for stereotactic radiosurgery, and sometimes there can be one lesion and sometimes there can be up to 78 lesions," he said. "This is incredibly time-consuming, but not all that difficult to actually do."
Image automation
AI could enable an automated system for performing a cardiac MRI scan, for example.
AI software developer HeartVista, for example, has developed AI-based software that can basically run a scanner, look at the images, and then adjust the planes so that a standardized exam is always acquired, according to Zaharchuk.
"I think this is really important when we think about all of the beautiful algorithms down the road and remember that they're incredibly dependent on [images] being acquired in the proper manner, and perhaps acquired in the proper manner across sites," he said. "This is definitely something to think about, and a very exciting area to focus on for AI in the future."
Image reconstruction
AI can really add value by making MRI go faster, Zaharchuk said. Progress has been made in the goal of getting MRI scans down to 10 minutes, but current barriers remain.
For example, fancy time-saving sequences may only be available on the latest scanners, or they may need a "key" from the vendor to turn it on.
"This may be surprising to a research audience, but one of the things I've found is that radiologists and technologists can be resistant to change," he said. "So we really do have to ask the question, 'Do the radiologists really want more scans to read?' And be cognizant of this as we're developing faster and faster algorithms."
Some questions/issues still need to be addressed for the use of deep learning in image reconstruction, including:
- The trade-offs of using k-space versus image domain space
- Determining the best loss function and assessment methods
- Pixel-wise mean-squared error loss is easier to optimize but can lead to blurry images
- Relevance of adversarial attacks
- Potential loss of clinically relevant information
However, many groups, including academic researchers and companies, have shown the ability of deep learning to reduce scan times, while maintaining equivalent image quality, he said. The technology can also be beneficial for denoising of functional exams such as diffusion-weighted imaging and diffusion tensor imaging, as well as for producing "superresolution" MRI exams, according to Zaharchuk.
AI could also enable higher-quality images on scanners with lower field strengths.
One-minute MRI?
Getting MRI exams down to one minute would be even more transformative for the modality than a 10-minute scan, according to Zaharchuk. For example, Stefan Skare, PhD, and his group at the Karolinska Institute in Sweden have been developing a one-minute full-brain MRI technique.
One-minute MRI would offer many benefits, including for quickly triaging normal versus abnormal cases; abnormal patients could then come back for a longer study. This could replace CT in the emergency department, and perhaps enable claustrophobic people to tolerate the shorter exam, Zaharchuk said.
"To really think outside of the box, think of MR as a walk-in service," he said. "When you get a prescription for a chest x-ray from your doctor, you don't schedule a time. You just take your prescription, go to the lab, and just walk in. If you could do MRI this fast, maybe this paradigm is also possible for MR."
Postprocessing applications
There are a number of potential AI applications in MRI postprocessing, including co-registration/normalization, segmentation, and standardization/harmonization, Zaharchuk said.
Co-registration is critical for many tasks, particularly longitudinal analysis. It's also useful for dynamic time-courses where motion is an issue, he said.
"I think most people would agree that existing methods are laborious and somewhat time-consuming and prone to failures," he said. "The ability of using deep learning-based systems for co-registration is really exciting long-term. And when this gets combined with segmentation, it gets very interesting."
Similarly, segmentation -- identifying the borders of lesions or anatomical structures -- is also critical for many tasks. Existing methods are also laborious and time-consuming, and having radiologists draw regions of interest just isn't feasible on a long-term basis, he said.
"Also, the potential for prediction -- looking at lesions in a current time and predicting perhaps what they will look like in the future -- might be valuable," he said.
For example, Zaharchuk's group at Stanford has developed a deep-learning algorithm that outperforms the current clinical standard method for predicting the probability of future infarction in acute ischemic stroke patients. Deep learning also shows much potential for improving quantitative imaging, such as in quantitative susceptibility mapping, he said.
Generalizability concerns
AI algorithms can perform differently when segmenting MR images from scanners from different vendors. However, research by Wenjun Yan et al published on July 1 in Radiology: Artificial Intelligence showed that a method that adapts to results from the different manufacturers could yield improved generalizability.
"So I think one of the questions moving forward is, should we be training deep-learning models for every different scanner, or should we be trying to make all scanners look the same and train individual deep-learning models?" Zaharchuk said.
AI postprocessing applications need to be robust to scanner type, technologist errors, and motion, according to Zaharchuk. They also need to be tightly integrated into the PACS software if radiologists are expected to use it in real-time. Questions also remain about how these applications will be commercialized, he said.
Image interpretation
Zaharchuk noted that an AI-based system was recently found to yield performance comparable to academic neuroradiologists in diagnosing 19 common and rare diseases on brain MRI exams.
But will autonomous AI show up in reading rooms anytime soon? In July, the American College of Radiology and the RSNA sent a letter to the U.S. Food and Drug Administration (FDA) stating their belief that autonomous AI -- AI used without radiologists in the loop -- should not be implemented at this time due to safety concerns.
"It will be very interesting to see how the FDA handles [autonomous AI technology]," he said. "But as an adjunct to radiologists, these [types of] techniques may find use in the near future."
The concept of population-based imaging AI has also drawn interest for identifying information on images that isn't immediately important but carries prognostic value, according to Zaharchuk. These findings could include coronary calcium scoring, lung fibrosis, white-matter hyperintensities, vertebral body fractures and osteopenia, and liver steatosis.
Algorithms could run in the background and refer patients for risk adjustment, Zaharchuk said.
New and different applications
Deep learning could be used to improve patient safety by enabling lower or even no doses of gadolinium-based contrast agents, for example. In addition, AI could be used for modality transformation, such as generating pseudo-CT images from MRI data to provide MR attenuation correction in a PET/MRI reconstruction pipeline, he said.
Also, a deep-learning algorithm could be used to predict, for example, how cerebral blood flow would be measured on PET by analysis of MRI. Furthermore, AI also shows promise for enabling zero-dose FDG imaging, combining imaging and clinical data to assist radiologists, Zaharchuk said.