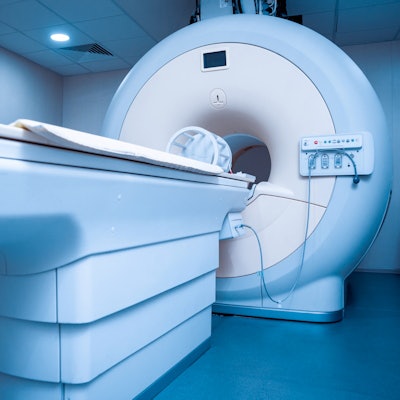
Artificial intelligence (AI) can yield valuable improvements across all elements of the MR imaging chain, according to a May 15 presentation at the International Society for Magnetic Resonance in Medicine (ISMRM) annual meeting.
For example, AI/machine-learning technology can enhance how scans are prescribed and can automate image acquisition, noted Dr. Shreyas Vasanawala, PhD, of Stanford University. Vasanawala is director of MRI at Stanford Children's Health and conducts research into developing faster and quantitative MRI methods.
When applied during MR image reconstruction, AI can improve image quality or speed up exam times, he told the ISMRM 2021 audience. What's more, AI can also detect and correct image artifacts, as well as perform image processing tasks such as segmentation.
Despite substantial technological development, AI hasn't been widely adopted yet by hospitals -- a delay partly due to the slow and difficult implementation process. Although the focus has been on the models themselves, an AI model in and of itself does not equate to a clinical application, Vasanawala said in his presentation on how to translate machine learning into the clinic.
That requires integrating the models -- and often a viewer for the models -- into the workflow. If not, the AI model is unlikely to achieve significant traction in the clinic.
"The main challenge here is that there isn't really a great platform that allows for translating these AI models into actual deployment and clinical applications," he said.
In spite of these barriers, there are some great opportunities for clinical deployment of AI in MRI, according to Vasanawala. For example, there are multiple efforts underway to automate scan prescriptions, leveraging AI or machine-learning approaches to significantly speed up image acquisitions.
In image reconstruction, AI can yield enhanced image quality with less noise, faster scans, and perhaps even greater quantitative accuracy, Vasanawala said.
"Vendors are working on these developments actively as we speak," he said. "But currently, this has not hit the mainstream in terms of products that are actually present for image reconstruction that leverage machine learning or AI approaches."
The use of AI in a reconstruction is also a hot area in the research setting, Vasanawala noted. At Stanford, deep learning-based reconstruction technology has been integrated, for example, into daily clinical practice to provide much faster cine imaging for cardiac MRI exams.
"Once these images come back through this deep-learning reconstruction algorithm, they can be passed to another algorithm for postprocessing," he explained.
AI can also be useful for detecting image artifacts, which can cause significant problems with image quality. With help from AI, MR technologists could receive real-time feedback on image quality and make any adjustments before patients leave the scanner.
Stanford is engaged in a number of efforts to integrate AI models that detect artifacts right at the scanner. As an example, researchers are collaborating with GE Healthcare on a scanner interface that displays automatically generated image quality scores on the fly, he said.
"And if the scores fall below a certain threshold, then you can have feedback that goes to technologists, where it can request for images to be repeated, or even repeated but with specific changes made to parameters," Vasanawala said.
Once image artifacts are detected, AI can even help to correct them prospectively, he said. Software from HeartVista, for example, can identify motion artifacts during scanning and then automatically repeat individual slices. Stanford researchers have also applied deep-learning algorithms to automatically correct artifacts for applications such as cardiac and abdominal 4D flow MRI, as well as to correct artifacts caused by field inhomogeneity.
Of the other elements in the imaging chain, image processing has generated the most attention and the most actual commercial products. With the plethora of available options in the marketplace, it can be challenging to figure out how to implement them in your clinical practice, according to Vasanawala.
"You have choices of whether these get integrated at an [electronic medical record] level or a PACS level or a dictation system level," he said.
The University Medical Center Utrecht in the Netherlands addressed this implementation challenge by developing a vendor-neutral AI deployment infrastructure, Vasanawala said. Called IMAGR, the infrastructure has two components: a portal and a server. Users log into the portal and utilize the AI processing tools such as segmentation.
"This provides a nice environment in which multiple different types of AI algorithms can all be deployed, and you're not fixed into one particular vendor's infrastructure," he explained.
Stanford researchers have also developed another approach for facilitating the use of AI in postprocessing. Called deep open-source medical image analysis (DOSMA), the AI-powered image analysis library includes components for data input and output, algorithm hosting, and results visualization, according to Vasanawala. It also interfaces with PACS. DOSMA will be presented at the ISMRM meeting during a software demonstration tutorial on May 18 at 3 p.m. Eastern time.