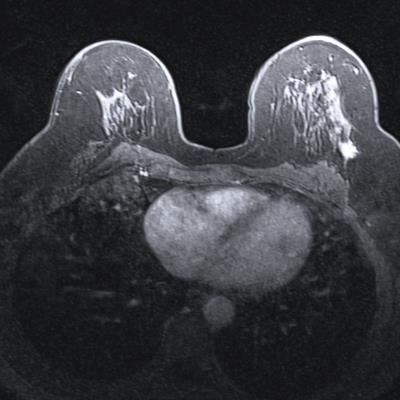
Using a nontraditional approach to training a deep-learning algorithm yielded higher specificity for classifying lesions found on breast MRI in a study published online June 6 in Academic Radiology.
Researchers from Columbia University Medical Center used weak supervision -- a method of training deep-learning models based on labels of image slices instead of manual pixel-by-pixel annotations -- to train a breast MRI lesion classification algorithm. In testing, the model yielded high specificity and an area under the curve (AUC) of 0.92.
"This approach may have implications on both workflow by making it more efficient to generate large datasets as well as potential use for breast MRI interpretation with high specificity," wrote the authors, led by Michael Liu.
Large, well-annotated datasets are typically needed in order to adequately train neural networks. However, using training images that are labeled at the slice level avoids the tedious pixel-by-pixel annotation of a region of interest (ROI) traditionally required to assemble a sufficiently sized dataset, according to the researchers.
"In addition to improving workflow, this approach has the added benefit of teaching the network imaging features of normal anatomy and benign enhancement patterns of the breast," the authors wrote.
In their study, Liu and colleagues sought to determine if a weakly supervised approach could enable the algorithm to learn from the whole image, instead of just from a delineated ROI within the image. They also wanted to assess the feasibility of this method for improving the specificity of breast MRI, which is known for having high sensitivity but also a high false-positive rate.
The researchers assembled a dataset of 438 breast MRI studies, including 307 from their institution as well as 131 cases from nine different U.S. institutions downloaded from the Investigation of Serial Studies to Predict Your Therapeutic Response with Imaging and Molecular Analysis (I-SPY TRIAL) database.
They then combined 278,685 image slices from these studies into 92,895 three-channel images. Of these, 79,871 (85%) were used for training and validation of their convolutional neural network (CNN). The remaining 13,024 images (15%) formed a testing dataset and included 11,498 benign images and 1,531 malignant images.
The algorithm yielded the following performance on the test set.
Performance of breast MRI deep-learning algorithm | |
AUC | 0.92 |
Sensitivity | 74% |
Specificity | 95.3% |
Accuracy | 94.2% |
"It is feasible to use a weakly supervised based CNN to assess breast MRI images with a high specificity," the authors concluded.
The weakly supervised training method provides a number of benefits, including facilitating compilation of larger datasets by obviating the need for manual pixel-level annotation, according to the researchers. In addition, the technique can decrease the subjective bias that can occur when human readers manually identify the boundaries of a ROI, they said.
"Thirdly, instead of just evaluating the ROI which often contains the breast tumor or a discrete benign lesion, the whole slice of the MRI images is evaluated by the network," Liu et al wrote. "This approach mimics a real-life clinical practice, incorporating an analysis of a full range of benign findings such as [background parenchymal enhancement] that the network can learn as benign enhancements."