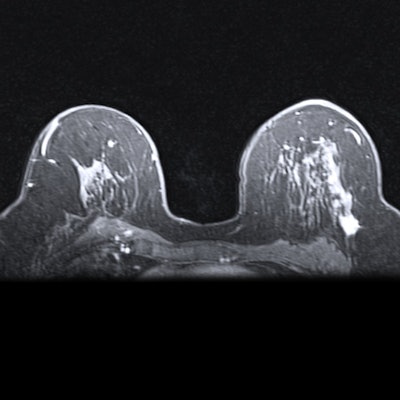
Prediction models based on clinical characteristics could help reduce false positives in women with dense breasts who undergo supplemental breast cancer screening with MRI, according to a study published August 17 in Radiology.
Researchers led by Dr. Bianca den Dekker from Utrecht University in the Netherlands said the finding could help bring supplemental screening MRI for women with dense breasts one step closer to successful implementation.
"Reduction of the false-positive recall rate is an important issue when considering the use of breast MRI as a screening tool," den Dekker told AuntMinnie.com.
Women with dense breasts are at higher risk for developing breast cancer than women with average breast density. MRI is touted as a useful addition to mammography screening in women with dense breasts, since dense breasts reduce the sensitivity of mammography.
However, MRI's prized high sensitivity can also detect benign lesions that otherwise would have gone unnoticed, leading women to get recalled for additional work. This means repeat MRI scans, targeted ultrasound, and biopsy. The researchers said this additional work can cause anxiety for patients, increase healthcare costs, or lead to biopsy-related complications.
"The need for additional investigations places a burden on both patients and the overall healthcare system," den Dekker said.
The team wanted to find out if prediction models could decrease false-positive rates. One model was based on all collected clinical characteristics and MRI findings, which the team called a full prediction model. The second model was solely based on readily available MRI findings and age.
The study authors looked at data from 454 Dutch women from the randomized controlled DENSE-Trial who had a positive MRI result in a first supplemental MRI screening round. The participants were asked to complete questionnaires on health and risk factors, such as personal and family history of breast cancer, body mass index, and menopausal status, among others.
Out of these, 79 women were diagnosed with breast cancer, meaning that 375 women had false-positive MRI examinations.
The researchers said that out of the 328 participants who had complete data, 64 had breast cancer and 264 had false-positive findings. With the full prediction model, a total of 120 of 264 (45.5%) false-positive findings could have been identified as false-positive cases without missing any cancers. The full model could have also prevented 21.3% of benign biopsies and had an area under the curve of 0.88, the team added.
The model based on only readily available MRI findings and age meanwhile could have prevented 35.5% of false-positive MRI screenings and 13.0% of benign biopsies. It also had an area under the curve of 0.84.
den Dekker said the team was "positively surprised" at the performance of the full prediction model.
While the full model showed promise, researchers said implementing it in clinics would need a "substantial" amount of time, money, and effort to collect clinical characteristics in screening practice.
"The amount of missing data, even within the context of a prospective randomized controlled trial, reflects the difficulty in collecting these characteristics and raises questions about the feasibility of such a model in daily practice," the study authors wrote.
The model solely based on readily available MRI findings and age, meanwhile, could be implemented relatively easily, they added.
den Dekker said the next step is to perform validation studies using data from different populations, including how the prediction models perform in subsequent screening rounds.
"As incident screening rounds have a much lower false-positive rate, separate models may have to be created," she told AuntMinnie.com.