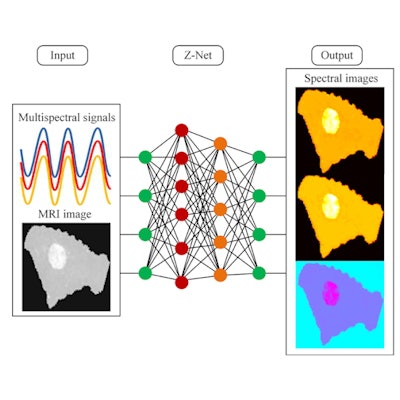
A new deep-learning algorithm shows promise for improving breast cancer detection by melding near-infrared spectral tomography (NIRST) and MRI data, according to a study published February 24 in Optica.
The algorithm is called Z-Net and combines NIRST with contrast-free MRI to "overcome a major hurdle in multi-modality imaging by allowing images to be recovered in real-time," according to a team led by Jinchao Feng, PhD, of Beijing University of Technology in China.
"The near infrared spectral tomography and MRI imaging platform we developed has shown promise, but the time and effort involved in image reconstruction has prevented it from being translated into the day-to-day clinical workflow," co-author Keith Paulsen, PhD, of Dartmouth College in Hanover, NH, said in an Optica statement. "Thus, we designed a deep-learning algorithm that incorporates anatomical image data from MRI to guide NIRST image formation."
NIRST produces data on clinical markers such as oxy- and deoxyhemoglobin, water, and lipid, and imaging components like scatter that help distinguish normal breast tissue from cancer. Noncontrast MRI-guided NIRST could prove an effective alternative to dynamic contrast-enhanced MRI, which some consider the most sensitive breast cancer detection method, but which requires intravenous contrast, Feng's team noted. However, NIRST needs refinement, and utilizing deep learning to reconstruct the images can help.
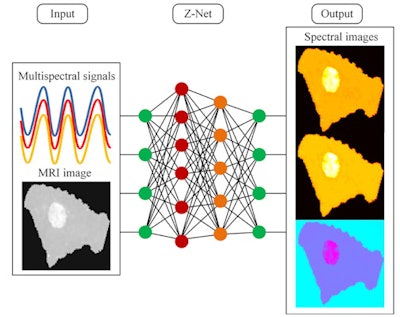
"The Z-Net algorithm reduces the time needed to generate a new image to a few seconds," Feng said. "Moreover, the machine learning network we developed can be trained with data generated by computer simulations rather than needing images from actual patient exams, which take a long time to collect and process into training information."
Feng and colleagues developed and trained the algorithm, then applied it to MRI-guided NIRST data they collected from two breast imaging exams (one showing malignancy, and one benign). They compared Z-Net's performance to another network architecture, Y-Net, and found that Z-Net required 43% fewer training parameters and 46% less training time -- without negative effect on reconstruction performance.
Training parameters and time for two deep-learning algorithms | ||
Metric | Y-Net | Z-Net |
Number of parameters | 6.09 | 3.48 |
Training time in hours | 7.2 | 3.9 |
Most importantly, the algorithm was able to generate images that distinguished between malignant and benign cases by highlighting total hemoglobin concentration (HbT) and water differences, the investigators reported. For example, in one of the cases, "HbT contrast between the suspicious mass and normal tissue was 1.05, suggesting that the lesion is benign," the group wrote. "Pathological analysis confirmed later that the abnormality was a fibroadenoma."
Z-Net could improve not only breast cancer detection but the detection of other cancers as well, Paulsen said in the Optica statement.
"Z-Net could allow NIRST to become an efficient and effective add-on to non-contrast MRI for breast cancer screening and diagnosis because it allows MRI-guided NIRST images to be recovered in nearly real time," he said. "It can also be readily adapted for use with other cancers and diseases for which multimodality imaging data are available."