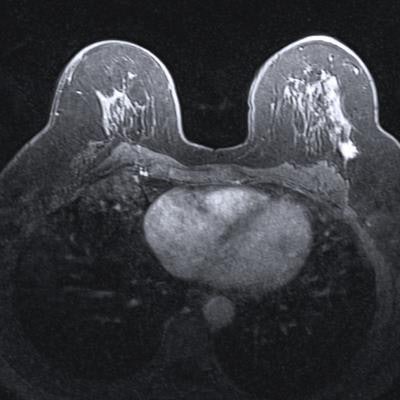
An artificial intelligence (AI) algorithm could triage 20% of screening breast MRI exams as normal without missing any cancers, say researchers from New York City. If used in clinical practice, AI could make breast MRI screening more efficient.
In a presentation from the International Society for Magnetic Resonance in Medicine (ISMRM) meeting in London, researchers from Memorial Sloan Kettering Cancer Center in New York City discussed how they developed a deep-learning model designed to triage normal screening breast MRI exams to a special worklist requiring only abbreviated review by a radiologist.
In testing, the algorithm performed well and would have yielded an estimated 20% time savings for the radiologists, according to presenter Arka Bhowmik, PhD.
"We developed a deep-learning tool that triages 20% of the normal cases to an abbreviated worklist without missing any cancers in the test set," he said.
Breast MRI is used for breast cancer screening in high-risk patients, but more than 98% of these exams are normal. In the study presented at ISMRM 2022, the researchers sought to utilize AI to triage completely normal exams to an abbreviated radiologist worklist; they also compared the algorithm's performance to that of fellowship-trained radiologists. They also wanted to estimate projected time savings from the use of AI.
The researchers developed their deep-learning model based on using the BI-RADS system to provide AI training labels. BI-RADS 1 cases were deemed to have no image findings, while the remaining BI-RADS scores (2-6) were considered to have image findings.
The researchers retrospectively gathered 16,020 contrast-enhanced axial breast MRI exams performed at their institution in 8,330 patients between 2013 and 2019. Of these, 12,911 (80%) exams were used for training, 1,627 (10%) were used for validation, and 1,482 (10%) were set aside for testing. In addition, 50 exams from the test set were randomly chosen for a reader study.
The test set included 1,467 cancer-free exams and 15 with cancer. The AI algorithm detected all 15 cases with cancer and triaged 20% of the cancer-free exams to an abbreviated interpretation worklist for a radiologist. The remaining 80% of exams were triaged for full interpretation by a radiologist.
The researchers calculated total projected reading time under this paradigm would have dropped from 148 hours to 119 hours, a time savings of 20%.
In a reader study comparing the performance of radiologists with the AI algorithm, 42 of the 50 MRI exams were cancer-free and eight included cancer. All eight cancer exams were identified by both the radiologists and the AI algorithm.
Of the 42 cancer-free exams, nine were triaged to an abbreviated worklist for radiologists and 33 were triaged to full radiologist interpretation. The radiologists dismissed 39 (92%) of the 42 cancer-free exams and flagged three (7%) for biopsy.
In the next phase of their work, the researchers are now performing a multi-institutional validation of the algorithm. They are also developing a more generalized end-to-end algorithm that includes an initial breast segmentation step, according to the authors.