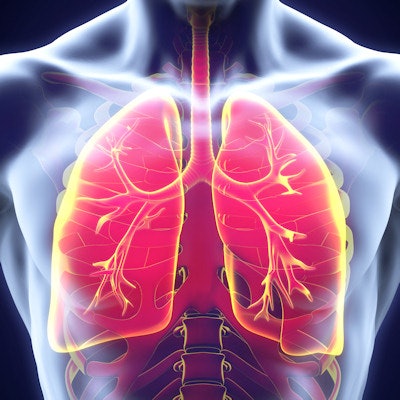
Four-dimensional CT images can improve radiation therapy planning in the upper thorax and abdomen, but motion artifacts often make the images nondiagnostic. New algorithms that provide deformable registration and respiratory motion simulation clear up the picture, researchers concluded in the International Journal of Computer Assisted Radiology and Surgery.
A study team from China and the U.S. built an algorithm consisting of two main stages: deformable image registration and respiratory motion simulation. The algorithm used a block-matching method to register each 4D CT phase image with the breath-holding CT image. The respiration motion of the lung was then recovered from a displacement vector field. Results showed that mean spatial error fell to 1 mm from 4.6 mm.
"The proposed method identified and reduced artifacts accurately and automatically, providing an alternative way to analyze 4D CT image quality and to correct problematic images for radiation therapy," wrote Min Li from Nanjing University of Science and Technology in China and MD Anderson Cancer Center, along with colleagues from other institutions in China and the U.S. (Int J Comput Assist Radiol Surg, February 14, 2017).
A moving target
Respiratory motion hampers the delivery of radiation therapy to the thorax and upper abdomen by creating uncertainty in the shape, volume, and location of tumors, the authors wrote. Four-dimensional CT addresses this issue by acquiring a series of 3D images at different moments in the patient's breathing cycle, thereby visualizing tumor motion. As a result, 4D CT, either cine or helical, is being used widely in radiation treatment planning and delivery, despite that images include significant artifacts that degrade image quality and represent anatomy inaccurately.
Artifacts in cine 4D CT images are primarily caused by imaging limitation from limited gantry rotation speeds and image reconstruction times, as well as by sorting errors from the irregular breathing patterns of patients. Many lung cancer patients are unable to breathe regularly, which makes motion artifacts even worse, even when patients are trained in breathing for the exam. In practice, human observation is routinely used to manually assess the extent of artifacts in 4DCT images and is the de-facto gold standard of artifact evaluation, Li and colleagues wrote.
In addition, observers may be not confident identifying artifacts because major image distortion makes artifacts readily visible, Li et al wrote, adding that human observation is subjective, time-consuming, and requires medical experts like physicians or physicists.
"The limitations of human observation necessitate the development of effective automated means of artifact identification and reduction," they wrote. But few studies have focused on this topic. So they created a respiratory model consisting of deformable image registration (DIR) and respiratory motion simulation to automatically identify and reduce artifacts caused by irregular respiratory motion in cine 4D CT images.
The registration established correspondence across the 4D CT images, thus enabling spatial and temporal lung motion to be modeled from a displacement vector field for respiratory motion simulation, the team explained. Using the model, artifact positions were located according to deviations between image points and their motion trajectories.
"Unlike approaches in many previous studies, the proposed algorithm focuses on identification and reduction of artifacts in sorted 4D CT images rather than retrospective analysis for 4D CT sorting process, which provides an effective means of analyzing 4D CT image quality and correcting aberrant data sections for radiation therapy," Li and colleagues noted.
Two-step process
For each patient, cine 4D CT images were acquired from patients under free-breathing using cine mode on a PET/CT scanner (Discovery ST, GE Healthcare) at the University of Texas MD Anderson Cancer Center in Houston. A respiratory tracking system (Real-Time Position Management Respiratory Gating System Varian Medical Systems) recorded respiratory signals for each patient.
The algorithm consisted of two main stages: deformable image registration and respiratory motion simulation. Each 4D CT phase image was registered to the breath-holding CT image using the block-matching method. Erroneous spatial matches were then removed by a least median of squares (LMS) filter and the full displacement vector field generated by the moving least squares interpolation.
The trajectory of the lung's respiratory motion was then recovered from the displacement vector field using a parameterized polynomial function, with fitting parameters estimated by combinatorial optimization. The four points that determined the optimal combination option was used as the most accurate positions of the voxel to estimate the optimal cubic polynomial fitting function. Using the combinatorial optimizations, they calculated a motion trajectory based on a cubic polynomial function for each component of motion. The distance between each image point and its motion trajectory was computed as the residual. Thus, each combinatorial option created 10 residuals corresponding to 10 different phases, they wrote.
The results showed sharply reduced mean spatial errors. The mean spatial error (standard deviation) was 1.00 mm (0.85 mm) after registration compared with 6.96 mm (4.61 mm) before registration. In comparison with human observation conducted by medical experts as the gold standard, the average sensitivity for artifact identification was 97%, specificity was 84%, and accuracy was 89%.
"The proposed method identified and reduced artifacts accurately and automatically, providing an alternative way to analyze 4D CT image quality and to correct problematic images for radiation therapy," Li and colleagues wrote.
The researchers believe there were a number of advantages to using their algorithm.
"In clinical settings, human observation is widely used for artifact identification," they wrote. But this lacks guidelines, making artifact quantification difficult on visual inspection. The method in this study "provides an alternative means of detecting artifacts -- an automatic and reproducible process that does not involve subjective intervention and is likely to be more reliable than human observation."
Among the limitations, high-quality 3D images at breath-holding are required to capture the correspondence between images at different phases. Also, the CT value varies between phases; however, the current study maps the reference images without taking the CT values into account.
"The proposed method not only improves artifact detection and reduction, but also shows potential as a means of validating the quality of images in which the presence of artifacts has been corrected," Li and colleagues concluded.