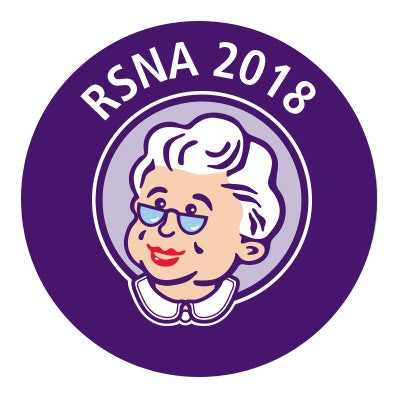
CHICAGO - Computer-aided diagnosis (CAD) technology helps radiologists of different experience levels better identify malignant thyroid nodules on ultrasound images, according to research presented on Monday at the RSNA meeting.
Dr. Feng Han, PhD, of Sun Yat-Sen University Cancer Center in Guangzhou, China, and colleagues evaluated the effect of CAD on radiologist performance via a study that included 1,065 thyroid nodules diagnosed between January 2013 and December 2017. Of these, 36% were benign and 64% were malignant. Four experienced radiologists and four radiology fellows analyzed the ultrasound images of the nodules, first without and then with CAD software.
Han's team evaluated the performance of the readers by tracking the area under the receiver operating characteristic curve (AUC), sensitivity, specificity, and interobserver agreement.
CAD improved the average performance of the eight readers, boosting the AUC value and increasing sensitivity and interobserver agreement, the researchers found. There was no statistically significant improvement in specificity without or with the use of CAD, however.
Reader performance for identifying thyroid nodules with and without CAD | |||
Performance measure | Interpretation without CAD | Interpretation with CAD | p-value |
AUC | 0.84 | 0.85 | < 0.0 |
Sensitivity | 86.4% | 87.5% | < 0.0 |
Interobserver agreement | 0.74 | 0.76 | < 0.05 |
CAD can help radiologists improve their sensitivity in the detection of thyroid malignancies, which translates into better practice, the researchers concluded.
"[CAD with ultrasound] could aid inexperienced radiologists in their diagnosis of thyroid malignancy," they wrote.
In a related presentation, Dr. Bin Zhang of First Affiliated Hospital of Jinan University, also in Guangzhou, shared results from a study of the performance of a machine-learning algorithm to diagnose thyroid cancer on ultrasound. The study included 2,064 thyroid nodules in 2,032 patients, meeting the following inclusion criteria:
- The nodule's maximum diameter was 2.5 cm.
- Patients had undergone partial or total thyroidectomy.
- Patients had undergone grayscale ultrasound and real-time elastography within a month before surgery.
- Patients had no previous thyroid surgery or percutaneous thermotherapy.
Zhang and colleagues used 60% of the cases to train the algorithm and 40% to validate it. They then calculated the AUC value of radiologist readers and compared it with results from the algorithm.
Random forest machine learning led to the best diagnostic model, they found. In fact, on grayscale ultrasound, the algorithm outperformed radiologist readers with an AUC of 0.92 versus 0.83.
The algorithm's success suggests a number of benefits for patients, according to Zhang.
"[This algorithm could help] reduce the risk of unnecessary surgery for benign conditions -- which, in turn, would reduce healthcare costs and psychological burden for patients," he concluded.