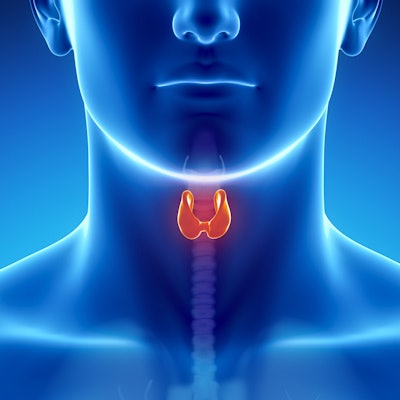
A deep-learning model developed by Chinese researchers can identify thyroid malignancies on ultrasound images, a study published December 21 in Computers in Biology and Medicine found.
Researchers led by Zhenggang Yu from Huaqiao University in Quanzhou found that by using an attention mechanism, their model can distinguish malignant from benign thyroid nodules with a high level of accuracy. They also highlighted the model's high speed.
"Young physicians and small hospitals with limited resources can benefit from using this method to assist with thyroid ultrasound examination diagnosis," Yu and co-authors wrote.
Early diagnosis of thyroid cancer with the aid of ultrasound imaging can lead to better treatment outcomes. However, this is dependent on the experience of the sonographer.
The researchers noted that while computer-aided diagnosis (CADx) techniques are available, their ability to differentiate between features seen in benign and malignant nodules is still limited.
Yu and fellow researchers wanted to propose another such method, but with an attentional mechanism with lightweight properties. They hypothesized that this could help identify nodules and distinguish malignant from benign findings by using a bounding box. They also highlighted that the model could do this without using much hardware while still being cost-effective.
The team tested the algorithm on data collected in 2020 and 2021 from a total of 1,921 thyroid ultrasound images from 1,357 patients. They looked at mean average precision (mAP) to evaluate performance and also assessed different intersection-over-union (IoU) thresholds, which measure the accuracy of an object detector on a particular dataset.
The researchers reported that the model showed good diagnostic results for benign and malignant thyroid nodules, especially for malignant nodules.
Performance of AI model for characterizing thyroid nodules on ultrasound | |||
All nodules | Benign | Malignant | |
Precision | 0.885 | 0.829 | 0.941 |
Recall | 0.851 | 0.838 | 0.863 |
mAP (0.5 IoU) | 0.89 | 0.868 | 0.911 |
mAP (0.5 – 0.95 IoU) | 0.501 | 0.478 | 0.523 |
The proposed model's mAP (with 0.5 IoU threshold) of 0.89 and an average speed of seven milliseconds, suggests high accuracy and rapid detection, according to the authors. They also highlighted that the model performs well with nodules of different sizes.
"In the clinical setting, it is possible to achieve a direct diagnosis of thyroid cancer once ultrasound images have been acquired, thus making the method practical," they added.
The study authors suggested that their model can aid inexperienced sonographers in this setting and can help them to free up their workloads. They added that it can also help avoid unnecessary invasive fine-needle aspiration.
However, the authors also called for better sample balance in testing the model. They wrote that their study was imbalanced between high- and low-risk nodules, which could result in poorer learning for the model.