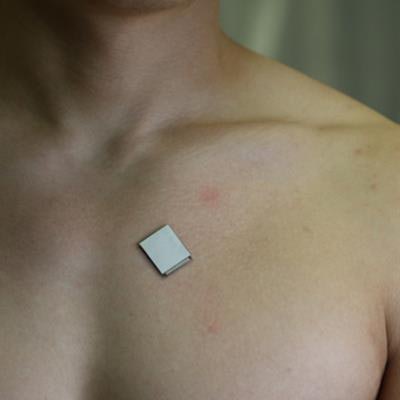
Researchers have developed a wearable patch and a deep-learning method that can assess the heart via ultrasound. They highlighted the device and its performance in a report published January 25 in Nature.
A team led by Dr. Hongjie Ju from the University of California, San Diego found that the method can successfully take cardiac images and transfer them into a digital network for assessment.
"This technology enables dynamic wearable monitoring of cardiac performance with substantially improved accuracy in various environments," Hu and co-authors wrote.
Continuous imaging of cardiac functions can help assess long-term cardiovascular health, detect acute cardiac dysfunction, and plan clinical management of critically ill or surgical patients. While noninvasive cardiac imaging methods exist, the researchers pointed out they have limited sampling capabilities and provide limited data, and they often rely on bulky devices.
Hu and colleagues wanted to investigate any progress on device designs and material fabrications that improve the coupling between an ultrasound device and human skin. They developed a deep-learning model that automatically extracts left ventricular volume from the continuous image recording on the wearable ultrasound device, providing waveform data of key cardiac performance guides such as stroke volume, cardiac output, and ejection fraction.
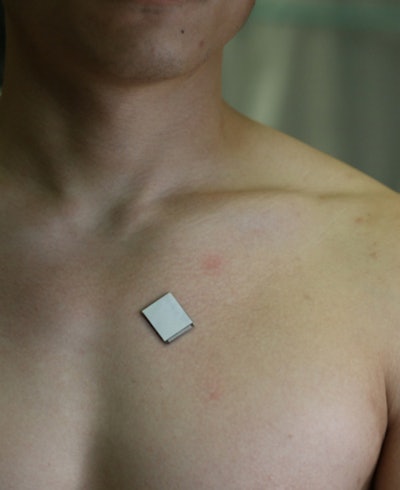
The device is the size of a postage stamp and connects directly to skin on the chest, adhering even during exercise. Hu's team used a piezoelectric one-to-three composite bonded with Ag-epoxy backing as the material for the transducers in the ultrasound imager, an approach that reduces risk and improves efficiency over other methods.
The investigators reported that the device was able to send and receive ultrasound waves which are used to generate a constant stream of cardiac images in real-time. They also used a wide-beam-compounding method to achieve the best signal-to-noise ratio and spatial resolutions.
For location accuracy, the agreements between the imaging results and the ground truths in the axial and lateral directions were high, at 96.01% and 95.9%, respectively. Additionally, grey values of inclusion images showed that the dynamic range was 63.2 dB, above the 60 dB threshold usually used in medical diagnosis.
Next steps for the technology include incorporating B-mode imaging, designing a soft imager, miniaturizing the back-end system, and working toward a general machine learning model that fits more subjects, the authors wrote.