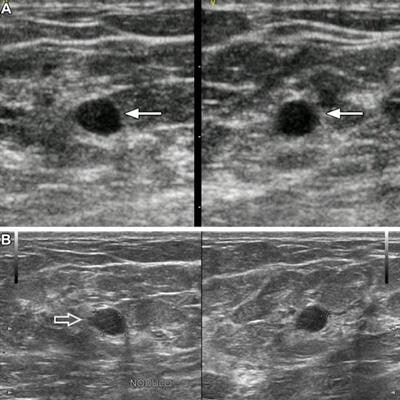
An artificial intelligence (AI) algorithm can accurately identify malignancies on portable ultrasound images of breast masses, according to research published May 2 in Radiology.
A team led by Dr. Wendie Berg, PhD, from the University of Pittsburgh and Magee-Womens Hospital found that their AI performed very well at identifying 96% to 98% of women with cancer no matter which ultrasound images were used, whether they be portable or standard images. The researchers suggested that this could be used in low-resource areas.
"This should improve access, health equity, and outcomes for women," Berg told AuntMinnie.com.
In many low-resource settings, including rural areas in the U.S., women may wait months to be seen, even when they notice a lump in their breast. Ultrasound of palpable breast masses requires appropriate equipment and trained professional staff for performance and interpretation.
Portable ultrasound, also known as point-of-care ultrasound (POCUS), has been shown to have value in these low-resource areas. Previous research suggests that this low-cost, accessible alternative has comparable performance to standard ultrasound and can be taught feasibly to nursing staff.
Berg and colleagues wanted to show that AI software (Koios DS) applied to breast ultrasound images obtained on low-cost portable equipment (GE HealthCare) and performed by minimally trained observers could accurately classify palpable breast masses for triage in a low-resource setting.
They tested their method at a hospital in Jalisco, Mexico, from 2017 through 2021. For the study, the team included data from 758 masses in 300 women who were analyzable by the AI. The women had an average age of 50 and the average largest lesion diameter was 13 mm. Of the total masses, 360 (47.5%) were palpable and 56 (7.4%) were malignant, including six ductal carcinoma in situ (DCIS).
The team reported that the AI correctly identified 47 or 48 of 49 women (96% to 98%) with cancer with either portable or standard-of-care ultrasound images. This included areas under the curve (AUC) of 0.91 and 0.95, respectively.
One circumscribed invasive ductal carcinoma was classified as probably benign with standard-of-care ultrasound, ipsilateral to a spiculated invasive ductal carcinoma.
Of 251 women with benign masses, 168 (67%) imaged with standard-of-care ultrasound were classified as benign or probably benign by the AI, compared with 96 (38%) on portable ultrasound (p < 0.001).
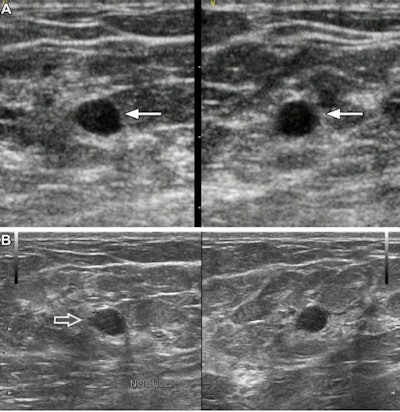
The researchers also found that AI performed significantly better with images obtained by a radiologist than with images obtained by a minimally trained observer.
"On images obtained by a radiologist on standard-of-care equipment, 80% of benign masses were recognized as such and could have been triaged to surveillance, as were 72% of benign masses scanned by minimally trained observers," Berg said.
She added that the AI had not been trained "at all" on the types of images obtained by the portable ultrasound scanner and was not as good at triaging benign masses on these lower-quality images. This included 52% by the radiologist and 33% by the minimally trained observers.
Berg also told AuntMinnie.com that healthcare resources can be better focused on women with cancer and reduce delays in diagnosis by reducing the number of women with benign lumps who need to be seen in central facilities and potentially have a biopsy.
"Newer ultrasound equipment is much better than what was used in this study, and we hope to conduct larger studies in low-resource areas of the U.S. and other countries," she said.
Dr. Priscilla Slanetz from Boston University concurred in an accompanying editorial about the potential of AI tools such as the one described by Berg et al for use in low-resource settings. She added with improvements in the technology and further education of onsite, nonradiologist providers, AI is "primed" to change how breast patients are cared for.
"Integrating AI into these lower-resource environments is yet another step that will lessen the burden of disease in these vulnerable patients who currently face major obstacles to care," she wrote. "The hope is that such steps will ultimately translate into lower mortality and better overall outcomes."