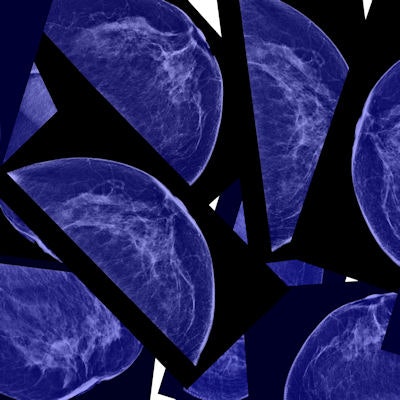
Artificial intelligence (AI) technology could help reduce unnecessary breast biopsies -- good news in an era when the focus is increasingly on curbing healthcare costs while also providing high-quality, appropriate care, according to a new study published online August 29 in Cancer.
As deep-learning algorithms are becoming ever more sophisticated and computing power continues to improve, some say it's inevitable that artificial intelligence tools will be increasingly integrated into radiology. And mammography will benefit, said lead author Stephen T. Wong, PhD, of Houston Methodist Hospital.
"Mammography in particular tends to lend itself to overdiagnosis and unnecessary biopsy, and artificial intelligence techniques such as machine learning and natural language processing can help reduce these by providing a more accurate clinical picture," Wong told AuntMinnie.com. "Obamacare calls for implementation of electronic health records, and once that raw data is in the digital archive, we can start mining it more cost-effectively to improve decision-making and patient care."
Proof of concept
Mammography reports tend to be heavy on free text, and, traditionally, mammographic imaging research has relied on manual analysis to convert this information into structured data, according to Wong and colleagues. But this kind of manual effort isn't realistic with large datasets (Cancer, August 29, 2016).
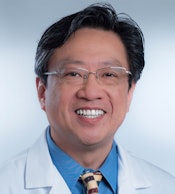
"Natural language processing has emerged as a possible solution with which to overcome the limitations of manual data abstraction and creates great opportunities in data mining of breast imaging," the authors wrote.
In a proof-of-concept study, Wong's team sought to investigate the usefulness of data mining and natural language processing (NLP) in defining a set of mammographic features that correlated with the molecular subtypes of breast cancer. The researchers developed an NLP software algorithm -- the Methodist Hospital text teaser, or MOTTE -- that extracts data from free-text mammography and pathology reports archived in the hospital's enterprise clinical data system.
"Various NLP techniques have been used to extract clinically useful information from mammography reports," they wrote. "However, to the best of our knowledge, the current study is the first to use NLP to characterize mammographic characteristics according to breast cancer subtype such that the correlation can be used to aid more robust clinical decisions."
Working with clinicians at Houston Methodist Cancer Center, Wong's team used the software to identify patients with BI-RADS 5 mammograms who underwent breast biopsy within three months of the date of their abnormal mammogram, between January 2006 and May 2015. A total of 717 patients fit the criteria; the researchers then used the software to search for cases of invasive carcinoma, identifying 543 patients.
These cases were further categorized by breast cancer subtype (estrogen receptor, progesterone receptor, and human epidermal growth factor receptor 2 status), body mass index, and breast tissue density. The algorithm's results were then confirmed by manual review, according to the team.
How did the software do? The algorithm showed that women with estrogen receptor-positive tumors were more likely to have spiculated margins, while those with human epidermal growth factor receptor 2 tumors were more likely to have heterogeneous calcifications. These results correlated with pathology reports of breast cancer subtype, the group wrote.
The study suggests that natural language processing may offer an accurate automated tool with which to create large-scale databases of breast imaging findings and malignancies -- which could help refine biopsy recommendations.
"This software intelligently reviews millions of records in a short amount of time, enabling us to determine breast cancer risk more efficiently using a patient's mammogram," Wong said in a statement released by Houston Methodist. "This has the potential to decrease unnecessary biopsies."
What about CAD?
The authors differentiate their proposed use of artificial intelligence from computer-aided detection (CAD) for mammography, which has been in use for years. Not only does MOTTE integrate more characteristics into its analysis than CAD algorithms do, but it is used after CAD has been employed.
"Mammography reports already contain any CAD data," Wong said. "But to make an accurate diagnosis, multiple types of data are needed, not just the image data CAD marks. Our algorithm incorporates more factors than CAD does, including those embedded in free clinical text and structured medical data."
In any case, radiology is a good candidate for the use of artificial intelligence, he said.
"Radiology has been one of the few medical fields that embraces technology, and that's smart," he said. "Artificial intelligence will help radiologists provide better service to referring clinicians and to patients."
Going forward, Wong's team plans to conduct future studies that will use the technology to further classify BI-RADS 4 patients by mining more than 10,000 free-text reports and image features.
"NLP algorithms can narrow the gap between the unstructured clinical reports and the detailed information extraction needed for data mining and decision-support tools," the group concluded.