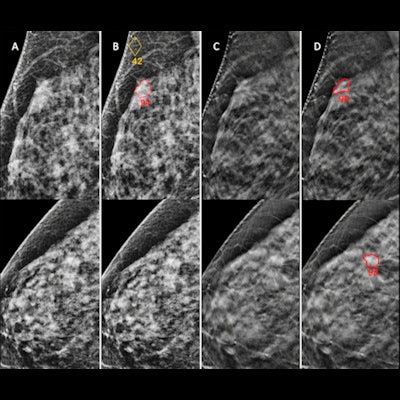
An artificial intelligence (AI) algorithm could sharply decrease radiologist workload for screening mammography and digital breast tomosynthesis (DBT) exams without hurting sensitivity, according to research published online May 5 in Radiology.
Researchers led by Dr. José Luis Raya-Povedano of Reina Sofía University Hospital in Córdoba, Spain, retrospectively compared a simulated autonomous AI triaging strategy with double reading on nearly 16,000 digital mammography and DBT exams. They found that AI could avoid the need for radiologist review in over 70% of DBT exams and digital mammography studies -- without negatively impacting sensitivity. What's more, an AI-based triage strategy for DBT exams was deemed superior to double reading of digital mammography, a capability that could facilitate adoption of DBT in breast cancer screening programs, according to the researchers.
"Given the increasing lack of expert breast radiologists as well as the increased workload associated with the introduction of DBT, new strategies potentially using AI could be necessary to maintain the cost-efficiency of screening programs," the authors wrote.
In an effort to evaluate how AI could potentially reduce radiologist workload without reducing cancer detection in different screening settings, Raya-Povedano and colleagues retrospectively collected 15,987 paired digital mammography and DBT exams from the Córdoba Tomosynthesis Screening Trial. These studies were acquired from January 2015 to December 2016 and initially interpreted with either single or double reading by radiologists.
The researchers then applied version 1.6 of the Transpara AI software (ScreenPoint Medical) to the studies and assessed the use of a simulated autonomous AI screening triage strategy in which the least suspicious exams would be read only by AI and then classified as normal. In addition, this strategy would also automatically recall exams scored as highly suspicious by AI but were not recalled by the radiologists.
They found that AI with DBT-based screening would enable a 72.4% lower workload for radiologists, a 16.7% lower recall rate, and noninferior sensitivity in comparison with double reading. What's more, it could also provide a 71.5% lower workflow for radiologists in digital mammography-based screening, as well as a 16.9% lower recall rate and noninferior sensitivity in comparison with double reading.
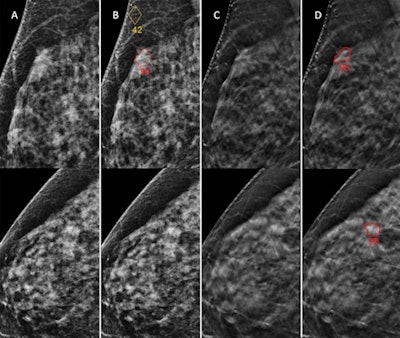
Moreover, AI could be used to facilitate a transition from digital mammography screening to DBT screening, thanks to a significant improvement in radiologist workload, sensitivity, and recall rate, according to the authors.
AI-based triage strategy for DBT exams vs. double reading of digital mammography | |||
Double reading of digital mammography exams without AI | AI-based triage and double reading of DBT exams | Improvement | |
Radiologist workload | 31,974 images (222 hours) | 8,830 images (156 hours) | 30%* |
Sensitivity | 67.3% | 84.1% | 25%* |
Recall rate | 5.1% | 3.7% | 27%* |
Raya-Povedano et al acknowledged several limitations to their study, including its reliance on a single site and single mammography and AI vendor. In addition, the study was retrospective in nature and utilized simulated AI scenarios, making it impossible to gauge the impact on radiologists' performance in a setting where they would only read the 30% most suspicious screening exams, according to the researchers. Further studies are also required to understand the clinical impact of using AI in screening when AI does not include prior information, they said.
"Finally, although our results suggest that no human reading of low-suspicion examinations would be the most optimal for the screening program cost-efficiency, further legal discussions would be needed to establish a framework where this strategy is safe for all the parties involved in screening," the authors wrote.