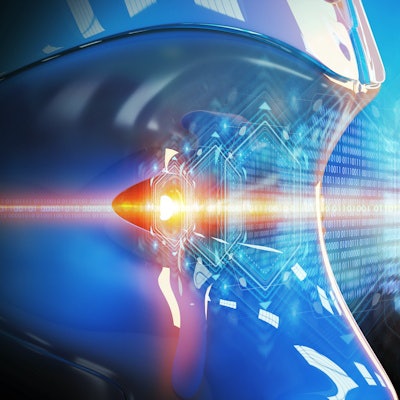
An automated process based on a deep-learning algorithm can identify scanned documents of radiology reports that need following up, according to research published online in the Journal of the American Medical Informatics Association.
A team of researchers led by Akshat Kumar and corresponding author Dr. Elmer Bernstam from the University of Texas Health Science Center at Houston developed an automated "pipeline" based on a transformer model, a deep-learning algorithm geared toward text analysis. In testing, their approach yielded high recall and a useful level of precision for automatically identifying scanned reports of mammograms, lung CT studies, and long-bone x-ray exams that contained clinically significant abnormalities.
"Our pipeline can identify abnormal reports with potentially useful performance and so decrease the manual effort required to screen for abnormal findings that require follow-up," the authors wrote.
Documents such as imaging reports that are scanned into the electronic health record (EHR) are often not categorized correctly, making them hard to locate and track. But even if they are correctly categorized after being scanned, it's laborious to manually review all clinically relevant documents, according to the researchers.
"Automatic extraction of pertinent documents could improve patient care by helping physicians identify and review important information about their patients," they wrote.
After training a fine-tuned version of the ClinicalBERT deep-learning model using typed/dictated reports from their institution's clinical data warehouse, the researchers then tested it on text from scanned documents extracted using optical character recognition.
"We chose mammograms/breast cancer, chest CT/lung cancer, and x-rays/long-bone fractures because they are among the most common imaging abnormalities associated with malpractice claims," they wrote.
Testing was performed on scanned documents including 120 mammograms, 140 chest CT studies, and 448 long-bone x-ray exams.
Accuracy and workload benefits from automated identification of abnormal findings on scanned imaging reports | |||
Long-bone x-rays | CTs | Mammograms | |
Area under the precision-recall curve | 0.75 | 0.90 | 0.92 |
Reduction in workload from use of the algorithm (when optimized for recall) | 42.5% | 30.5% | 83.5% |
Their approach could be used in multiple different scenarios, according to the researchers.
"Implementations prioritizing recall can be used in situations where there is a high priority to identify all abnormalities (e.g., screening incoming documents for pathologies)," the authors wrote. "Alternatively, lower recall could be acceptable to find patients eligible for particular research studies."
Furthermore, the algorithm's likelihood ratios could be used to prioritize documents for immediate review. What's more, the algorithm could identify reports with target abnormalities within the record of a patient with many radiology reports. Their pipeline could also be modified to classify scanned documents into granular categories such as definitely abnormal or probably abnormal, according to the researchers.
"In future work, we plan on creating better-automated methods for classifying our training data, perhaps using unsupervised machine-learning approaches to create a hybrid unsupervised-supervised pipeline or utilizing recent advancements from the field of N-shot learning," the authors wrote. "We will also test if our pipeline will generalize to other abnormalities."