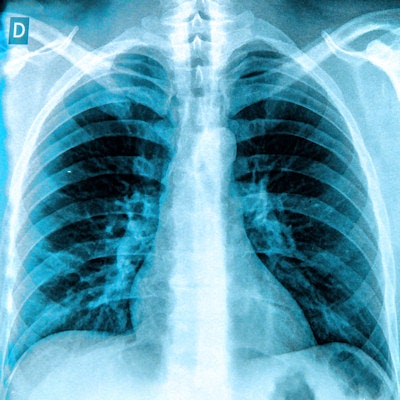
A computer-aided detection (CAD) system developed using deep-learning technology can perform an initial review of chest x-rays, helping radiologists to prioritize interpretation of cases that are more likely to be abnormal, according to a presentation last week at ECR 2017.
A team from La Fe Health Research Institute in Valencia, Spain, and its software developer spin-off Quibim has developed a CAD system based on convolutional neural networks (CNNs). The algorithm can produce more than 80% sensitivity and specificity for detecting abnormalities on chest x-rays.
"Our study improves or equals the results achieved by classifiers trained similarly in previous studies," said Belén Fos-Guarinos, an internship student with La Fe Health Research Institute's biomedical imaging research group. She presented the results during a scientific session at ECR 2017.
A demanding task
Reading chest x-rays is a demanding task for radiologists and can be very important medicolegally, Fos-Guarinos said. As a result, the group wanted to create a screening tool that could prefilter studies and assign a priority to abnormal exams, therefore facilitating workflow for reading these studies.
To accomplish that goal, they set out to design and develop a deep learning-based CAD system that could automatically perform initial screening of both normal and abnormal chest radiographs, according to Fos-Guarinos. They also wanted to explore the potential of CNNs for detecting chest pathologies.
In the first step of developing the CAD system, the researchers prepared the radiological database they would use to train and test the software. They gathered a subset of 2,255 posteroanterior DICOM chest x-rays from the Indiana University chest x-ray collection, which consisted of 868 abnormal exams and 1,387 normal studies.
The abnormal cases included 412 exams with opacity, 331 with cardiomegaly, 293 with atelectasis, 253 with a nodule, and 144 with a pleural effusion. The software was trained on 1,800 of the 2,255 images (80%); the remaining 455 images were used to test and evaluate the system.
To extract image features from the different groups of images, the researchers applied AlexNet, a convolutional neural network that was pretrained on the ImageNet image database. They used the Tesla K40 graphics processing unit accelerator from Nvidia to optimize computing performance.
"Once [the CNN] learns to extract [differentiating] features of different types of images, we use it to extract features that differentiate our two categories [of normal versus abnormal images], as would the human brain," she said.
Next, these automatically extracted features were used to train support vector machines, which provide classification of the images. The classifier initially determines if the study is normal or abnormal; images categorized as normal are displayed accordingly on the user interface. Images classified as abnormal, however, also go through an additional five classifiers to specifically assess for the presence of opacity, cardiomegaly, atelectasis, nodule, and pleural effusion.
Good performance
In testing, the system performed well overall, producing 83% sensitivity and 80% specificity for abnormal cases.
Performance of CAD system | ||||||
Overall detection of abnormal cases | Detection of atelectasis | Detection of cardiomegaly | Detection of pleural effusion | Detection of nodule | Detection of opacity | |
Sensitivity | 83% | 81% | 89% | 86% | 71% | 82% |
Specificity | 80% | 83% | 85% | 75% | 92% | 80% |
Accuracy | 82% | 82% | 87% | 80% | 82% | 82% |
Area under the curve | 89% | 90% | 91% | 91% | 85% | 88% |
"And [the software is] improving with more cases," Fos-Guarinos said.
AlexNet offers great potential in helping to get relevant results in screening of chest x-ray studies, she said.
"From now on, deep learning by CNNs has to be considered as the first candidate in any essential task of visual recognition," she said.