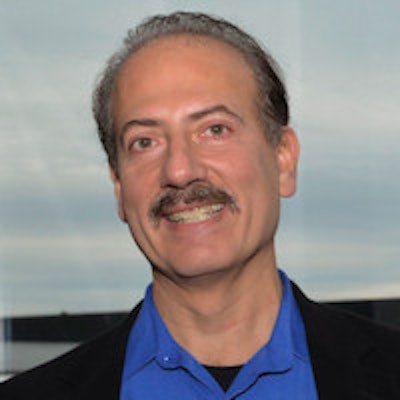
Artificial intelligence (AI) is all the rage right now, with most of the focus on using the technology for image analysis. But Dr. Eliot Siegel believes that AI has much more to offer, and he suggested several improvements at the recent International Society for Computed Tomography (ISCT) meeting.
Developers of artificial intelligence and machine-learning algorithms should move beyond analyzing images for signs of disease, and instead figure out ways to help radiologists do their jobs faster and more efficiently, noted Siegel, who is from the University of Maryland and the Veterans Affairs Maryland Healthcare System. If they don't, AI developers run the risk of going down the same path trod by other informatics technologies that once seemed revolutionary but fell short of expectations.
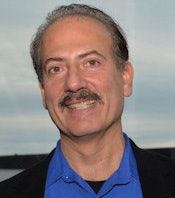
"There is a lot of skepticism from my colleagues about the added value of machine learning," Siegel said.
Defining AI broadly
If you define artificial intelligence broadly, the current crop of algorithms really only represent the latest iteration of a technology that's been around for some five decades, Siegel noted. He sees technologies such as computer-aided detection (CAD) and speech recognition as the first generation of artificial intelligence and machine learning in radiology.
CAD has been around since the 1990s, and it's still widely used today for mammography. However, in a recent survey only about 2% of radiologists said they always rely on CAD to provide an accurate diagnosis, and half said they never rely on CAD, Siegel explained.
"There's really an interesting mismatch between utilization of CAD for mammography and the level of reliance that we radiologists have on it," he told ISCT attendees. "The question is, are there lessons we can learn from mammography and CAD?"
The next generation of artificial intelligence should improve efficiency and productivity, increase accuracy and reliability, and do so at an affordable price. Siegel offered the following suggestions to make AI truly useful:
- It should measure things radiologists aren't already measuring. These could be features such as liver and pulmonary texture.
- It shouldn't constrain a radiologist's workflow. Some existing CAD models require studies to be sent to the cloud for processing and then be returned to radiologists. Instead, algorithms should be integrated into workflow.
- Its findings should be available on the radiologist's workstation. There have been thousands of AI and machine-learning algorithms developed over the years, but if they aren't easily accessible to radiologists at their workstation, then they probably won't be successful. "How can we take all these algorithms that are being written ... and be able to deliver them to the workstation?" Siegel asked.
- It shouldn't just act like a second reader. Radiologists haven't warmed to CAD because its focus on providing a second read duplicates what radiologists are already doing. But what if it offered tools such as color-coded probability maps of malignancy, or lesion tracking over time through prior studies? "I don't want the system to act as though it's never met the patient," Siegel said.
- It should tell us why, not just whether. Currently, CAD and AI algorithms are focusing on a binary decision: whether or not someone has disease. Instead, Siegel thinks it would be more useful if AI told radiologists why it marked a lesion or flagged a region of interest. Was it the lesion's size? Morphology? Density? All of this is information that a radiologist might find useful in coming to his or her own conclusion.
- It should provide quality feedback to radiologists. Siegel believes it would be useful to have an AI algorithm score the quality of his radiology reports, with respect to hedging and confidence, as well as improving communications and follow-up.
- It should learn from its own mistakes. Siegel provided the example of errors in speech recognition software, such as an application that renders "4.8" as "foreplay." Could AI be used to improve speech recognition by analyzing words the algorithm isn't sure about, or that don't make sense in a contextual way?
Finally, Siegel would love to see developers of machine learning and artificial intelligence algorithms shed their focus on image analysis and data quantification, and move into other areas that affect the work of radiologists. These could include automatic protocoling of CT and MRI studies, optimization of CT and MR image quality, follow-up on recommendations, synthesis of information from electronic medical records to present to radiologists, risk management, and more.
"Most of the discussion when we talk about machine learning and AI in radiology is focused on the images and findings themselves, but there are incredible opportunities that exist to be able to use machine learning to change our practice in so many other ways," Siegel said. "I think the most exciting and immediate applications are actually nonimaging applications."