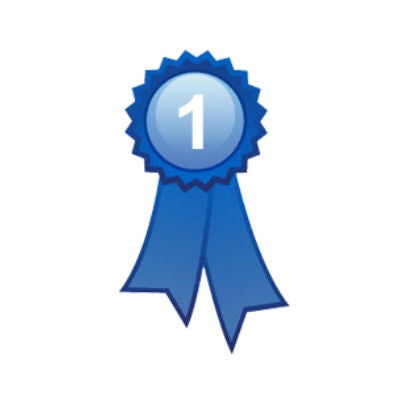
The use of chest radiography is continuing to grow around the world. Unfortunately, the number of healthcare professionals available to reliably report chest radiographs isn't increasing in tandem, said presenter Dr. Shalini Govil from Columbia Asia Radiology Group.
"This is partly due to the waning interest in x-ray reporting among radiologists and a lack of adequate training among physicians in the wards, emergency department, and intensive care unit," she said. "X-ray interpretation is also notoriously difficult with high false-negative rates and interobserver variability."
To address this challenge, Columbia Asia Radiology Group partnered with imaging AI software developer Qure.ai to evaluate a deep-learning algorithm for diagnosing chest radiographs. Importantly, the algorithm can generate explanatory "heat maps" that show radiologists the regions of the image that led to the diagnosis, Govil said.
"These heat maps enable radiologists to see through the computer's 'eyes' when validating the computer's diagnosis," she said. "This builds confidence in the machine's ability to evaluate something as complex as a chest radiograph and could potentially provide a means for providing feedback to the 'trainee computer.' "
The algorithm can reliably identify common abnormalities seen on chest x-rays such as consolidation, pleural effusion, cardiomegaly, fibrosis, and hilar adenopathy, according to Govil.
"It has the potential to markedly improve radiologist productivity and consign the albatross of 'unreported chest x-rays' to the annals of history," she said. "The algorithm can also serve as a means of triage and bring radiographs with critical findings to the top of the reporting pile. Perhaps, in the future, when confidence in machine diagnosis is high, these algorithms could be used in quality control to detect errors or help adjudicate radiology report discrepancies."
This paper received a Roadie 2017 award for the most popular abstract by page views in this Road to RSNA section.