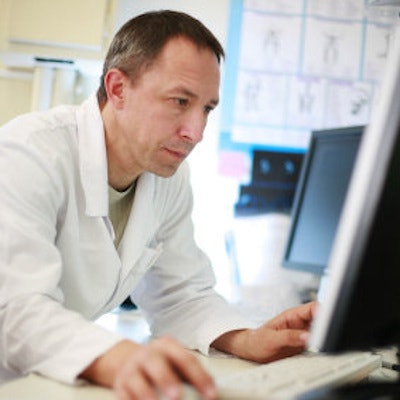
Success for artificial intelligence (AI) in radiology will be determined by its ability to increase diagnostic certainty, speed turnaround, yield better patient outcomes, and improve the work life of radiologists, according to an article published online February 4 in the Journal of the American College of Radiology.
AI could add value by discovering new knowledge and extracting more and better information from imaging examinations in order to achieve better outcomes for patients at lower cost, wrote the team of Massachusetts General Hospital (MGH) researchers led by Dr. James Thrall.
"For radiologists, adding value includes establishment of more efficient work processes and improved job satisfaction," they wrote.
The authors noted that research efforts for AI in imaging would benefit from national and international image sharing networks, reference datasets of proven cases for testing and comparing AI algorithms, criteria for standardizing and optimizing imaging protocols used in AI applications, and a common lexicon for describing and reporting AI applications.
While much of the initial research on clinical AI has focused on improving diagnostic accuracy, AI can also be used to address practical issues, according to the group. These opportunities include optimizing worklists to prioritize cases, preanalyzing cases in high-volume applications where observer fatigue may be a factor, extracting information from images that is not apparent to the naked eye, and enhancing the quality of reconstructed images.
Concern that radiologists will be displaced by AI is a potential barrier, but job loss is an unlikely outcome, according to the authors.
"Rather than replacing radiologists, the much more likely prospect is that the practice of radiology will beneficially assimilate AI methods, improving quality and efficiency similar to the experience with other digital imaging methods," they wrote.
Some key technical challenges also need to be addressed, such as figuring out how to establish the best source of truth for validating results, determining if processing speeds will be fast enough to be relevant for clinical practice, investigating whether protocol-tolerant AI programs can be developed, and exploring whether criteria can be established for determining if an AI program is valid for a given patient population.
AI's biggest limitation in imaging, however, may be the inherent limitations in defining normal versus abnormal in continuously variable biologic data, according to Thrall and colleagues.
"Investigators in AI will face this conundrum where nominal criteria for normal versus abnormal can be difficult to define when, for example, setting limits for organ sizes," they wrote.
The authors added that it's not yet clear what role AI will have in imaging or how the technology will affect radiologists.
"What is clear is that AI provides a promising new set of tools for interrogating image data that should be explored with vigor," they concluded. "The growing interest in AI in the imaging community bodes well for its potential leadership role."