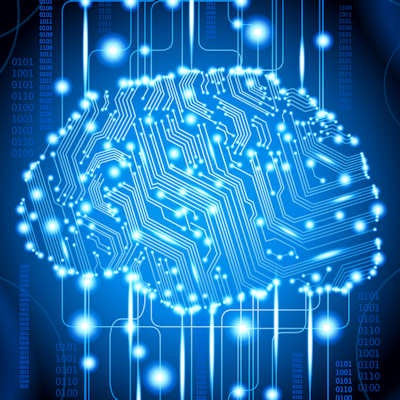
Artificial intelligence (AI) technology holds much promise for improving the quality of healthcare, but there are crucial ethical issues that need to be considered for the benefits of machine learning to be realized, according to a perspective piece published March 15 in the New England Journal of Medicine.
Implementation of AI in healthcare requires addressing ethical challenges such as the potential for unethical or cheating algorithms, algorithms trained with incomplete or biased data, a lack of understanding of the limitations or extent of algorithms, and the effect of AI on the fundamental fiduciary relationship between physicians and patients, according to a Stanford University team led by Dr. Danton Char.
All of these issues are also relevant to radiology, said Char, who is an assistant director at Stanford's Center for Biomedical Informatics Research and co-director of Spectrum, a research center funded by a U.S. National Institutes of Health (NIH) Clinical and Translational Science Award.
Unethical, cheating algorithms?
AI algorithms could be designed to perform in unethical ways -- as evidenced in nonhealthcare examples such as Uber's Greyball algorithm for predicting which potential passengers might be undercover law-enforcement officers. Algorithms can also be developed to cheat, such as when Volkswagen's algorithm enabled vehicles to pass emission tests by reducing nitrogen oxide emissions during tests.
Similarly, developers of AI for healthcare applications may have values that are not always aligned with the values of clinicians, according to Char and Stanford colleagues Nigam Shah, PhD, and David Magnus, PhD.
There may be temptation, for example, to guide systems toward clinical actions that would improve quality metrics but not necessarily patient care. Or these algorithms may be able to skew data provided for public evaluation when being reviewed by potential hospital regulators, according to the authors.
It's also possible to program clinical decision-support systems in a manner that would generate increased profits for their designers or purchasers, such as by recommending tests, drugs, or devices in which they hold a stake, or by altering referral patterns.
"The motivations of profit versus best patient outcomes may at times conflict," Char told AuntMinnie.com.
This perpetual tension of generating profit versus improving patient health must be addressed, because the developers and purchasers of machine-learning systems are unlikely to be the ones delivering bedside care, according to Char and colleagues.
Another issue is the danger of self-fulfilling prophecies. The underlying data used to train an algorithm can be incomplete or biased, and the functioning algorithm may then reflect these biases.
"If clinicians always withdraw care in patients with certain findings (extreme prematurity or a brain injury, for example), machine-learning systems may conclude that such findings are always fatal," the authors wrote. "On the other hand, it's also possible that machine learning, when properly deployed, could help resolve disparities in healthcare delivery if algorithms could be built to compensate for known biases or identify areas of needed research."
Blind faith or skepticism
In addition, physicians don't understand the limitations or extent of AI algorithms, creating the potential for blind faith or skepticism.
"Treating them as black boxes may lead physicians to over-rely or under-rely on AI systems," Char said.
The authors noted, though, that physicians who use machine-learning systems can become more educated about how these algorithms are constructed, the datasets they were trained from, and their limitations.
"Remaining ignorant about the construction of machine-learning systems or allowing them to be constructed as black boxes could lead to ethically problematic outcomes," they wrote.
Fiduciary relationship
AI also changes the dyadic, fiduciary relationship between the physician and the patient -- a relationship that has already been shifting in clinical medicine to one between a patient and an electronic health record system, Char said.
"Things like privacy are already difficult to guarantee," he said. "Implementing AI systems takes these [concerns] even further. What role and ethical duties do these autonomous systems have in the fiduciary relationship between a patient and their provider?"
With AI, the relationship will now be between the patient and the health system, according to the authors. Consequently, the adoption of machine-learning systems will require a reimagining of confidentiality and other core tenets of professional ethics.
"What's more, a learning healthcare system will have agency, which will also need to be factored into ethical considerations surrounding patient care," they wrote.
Ensuring ethical standards
The authors believe that the potential for bias and questions about the fiduciary relationship between patients and machine-learning systems are challenges that need to be addressed as soon as possible.
"Machine-learning systems could be built to reflect the ethical standards that have guided other actors in healthcare -- and could be held to those standards," they concluded. "A key step will be determining how to ensure that they are -- whether by means of policy enactment, programming approaches, task-force work, or a combination of these strategies."