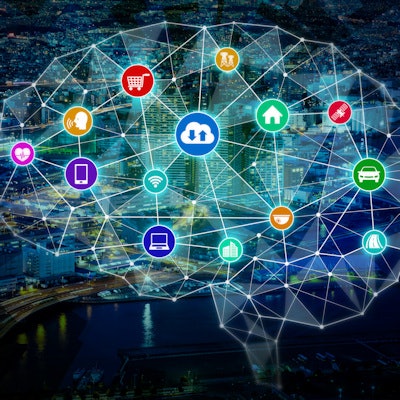
Radiology artificial intelligence (AI) algorithms must be properly validated on external image data before being used clinically for image analysis tasks. But most studies in the literature haven't performed this crucial step, according to research published in the March issue of the Korean Journal of Radiology.
After reviewing recent radiology AI studies in the literature, a team of researchers from South Korea found that only 6% reported testing their algorithms on external data -- i.e., images that were different from the ones on which they were trained. What's more, the few studies that did perform external validation still weren't designed adequately to determine readiness for clinical practice, according to the group led by senior author Dr. Seong Ho Park, PhD, and co-first author Dr. Hye Young Jang of the University of Ulsan College of Medicine in Seoul, as well as co-first-author Dr. Dong Wook Kim of Taean-gun Health Center and County Hospital.
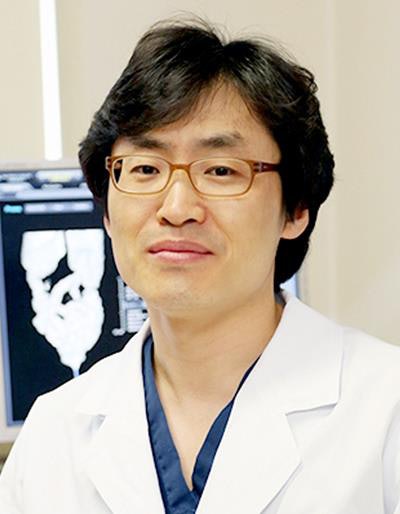
To properly perform external validation of deep learning-based algorithms for medical image analysis, researchers need to test these models on datasets that are sufficiently sized and collected either from newly recruited patients or from institutions other than those that provided the training data, according to the researchers. This process needs to adequately encompass all relevant variations in patient demographics and disease states encountered in the real-world clinical settings where algorithms will be applied, they said.
"Furthermore, use of data from multiple external institutions is important for the validation to verify the algorithm's ability to generalize across the expected variability in a variety of hospital systems," the authors wrote (Korean J Radiol, March 2019, Vol. 20:3, pp. 405-410).
To assess the design characteristics of radiology AI studies in the literature, the team searched the PubMed Medline and Embase databases to identify original research articles published between January 1, 2018, and August 17, 2018, that investigated the performance of AI algorithms used to produce diagnostic decisions by analyzing medical images. Next, they evaluated the eligible articles to determine if each study used internal or external validation for the algorithms.
For those studies that did perform external validation, the researchers reviewed the study design to determine if data were collected using three recommended criteria:
- With a diagnostic cohort design instead of a diagnostic case-control design
- From multiple institutions
- In a prospective manner
Only 31 (6%) of the 516 eligible published studies performed external validation of the algorithms, and none met the recommended criteria for clinical validation of AI in real-world practice.
"Readers and investigators alike should distinguish between proof-of-concept technical feasibility studies and studies to validate clinical performance of AI, and should avoid incorrectly considering the results from studies that do not fulfill the criteria mentioned above as sound proof of clinical validation," they wrote.
Acknowledging, however, that not every radiology AI research study is meant to validate real-world clinical performance, the researchers noted that they didn't intend to bluntly judge the methodological appropriateness of published studies.