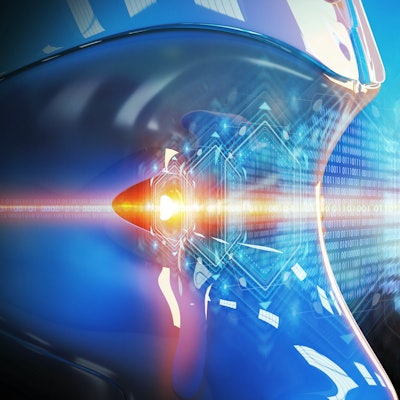
A meta-analysis of radiology artificial intelligence (AI) research found the technology had high overall accuracy in chest and breast imaging, but also widespread methodological and reporting issues that may preclude definitive assessments of clinical utility.
In a report published online April 7 in NPJ Digital Medicine, a team from Imperial College London shared their analysis of over 200 studies published on the use of deep-learning algorithms in breast or respiratory imaging applications. Although the models offered high overall diagnostic performance overall, the researchers cautioned that the research studies were also highly heterogeneous, with extensive variation in methodology, terminology, and outcome measures.
"While the results demonstrate that [deep learning] currently has a high diagnostic accuracy, it is important that these findings are assumed in the presence of poor design, conduct, and reporting of studies, which can lead to bias and overestimating the power of these algorithms," wrote first author Dr. Ravi Aggarwal, corresponding author Dr. Hutan Ashrafian, and colleagues.
The study is the latest to address the potential shortcomings of AI research in radiology, which have included the following:
- A recent study by another U.K. group that highlighted the flaws in COVID-19 AI studies
- A research letter detailing flaws in the geographic diversity of AI training sets
- A study sharing the risks of gender imbalance in datasets
- A report in 2020 that many medical imaging AI research studies were of poor quality and exaggerated claims
- A 2019 study reporting that most radiology AI studies lacked proper validation
In this meta-analysis, the researchers sought to quantify the diagnostic accuracy of AI in specialty-specific radiology applications, as well as to assess the variation in methodology and reporting of deep learning-based radiological diagnosis. After initially identifying nearly 12,000 abstracts for deep learning in medical imaging, Aggarval et al eventually winnowed the list down to 279 total studies, including 115 in respiratory medicine, 82 in breast cancer, and 82 in ophthalmology.
The researchers found high overall performance for radiology AI applications:
- Diagnosing lung nodules or lung cancer on chest x-ray or CT: Area under the curve (AUC) range = 0.864-0.937
- Diagnosing breast cancer on mammography, ultrasound, MRI, or digital breast tomosynthesis (DBT): AUC range = 0.868-0.909
The authors found high sensitivity, specificity, and AUC for algorithms in identifying chest pathology on CT scans and chest x-rays. Deep-learning algorithms on CT had higher sensitivity and AUC for detecting lung nodules, while chest x-ray algorithms produced higher specificity, positive predictive value, and F1 scores. In addition, deep-learning models for CT yielded higher sensitivity than those for chest x-ray in diagnosing cancer or lung mass.
In breast imaging, the researchers found generally high diagnostic accuracy -- and very similar performance by AI between modalities -- for identifying breast cancer on mammography, ultrasound, and DBT. Diagnostic accuracy was lower, however, for AI in breast MRI, perhaps due to small datasets and the use of 2D images, according to the authors. Utilizing larger databases and multiparametric MRI may increase diagnostic accuracy, they said.
Despite the results showing AI's high accuracy, it's difficult to determine if the algorithms are clinically acceptable or applicable, according to the researchers.
"This is partially due to the extensive variation and risk of bias identified in the literature to date," they wrote. "Furthermore, the definition of what threshold is acceptable for clinical use and tolerance for errors varies greatly across diseases and clinical scenarios."
The researchers found a large degree of variation in methodology, reference standards, terminology, and reporting. The most common variables included issues with the quality and size of datasets, metrics used to report performance, and validation methods, according to the authors.
The researchers offered five recommendations for improving the quality of future AI research:
- Availability of large, open-source, diverse anonymized datasets with annotations
- Collaboration with academic centers to utilize their expertise in pragmatic trial design and methodology
- Creation of AI-specific reporting standards
- Development of specific tools for determining the risk of study bias and applicability
- Creation of an updated specific ethical and legal framework