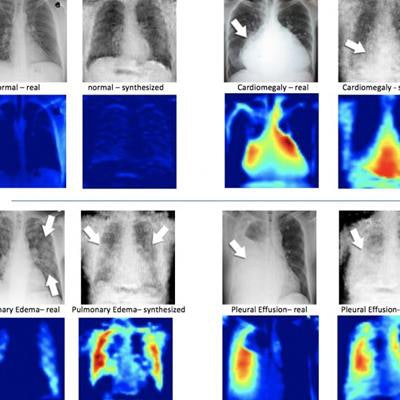
Researchers from Canada have developed an artificial intelligence (AI) algorithm capable of generating "artificial" x-ray images that look just like the real thing. The artificial images can be used to train other AI algorithms in analyzing real medical images.
The algorithm is designed to address one of the most vexing problems in the development of AI algorithms: the lack of image databases that are large enough to train algorithms in image interpretation in supervised settings. For example, the U.S. National Institutes of Health late last year took steps to address this problem by opening up a massive database of anonymized chest x-rays from actual patients for algorithm training.
Researchers from the University of Toronto's Machine Intelligence in Medicine Lab (MIMLab) are taking a different tack. They developed an algorithm called a deep convolutional generative adversarial network (DCGAN) that can create simulated x-rays which can then be combined into a database with actual x-ray images. The database can then be analyzed by other types of AI algorithms to determine whether an image is normal or shows pathology.
"In a sense, we are using machine learning to do machine learning," said Shahrokh Valaee, PhD, a MIMLab researcher who is leading the work.
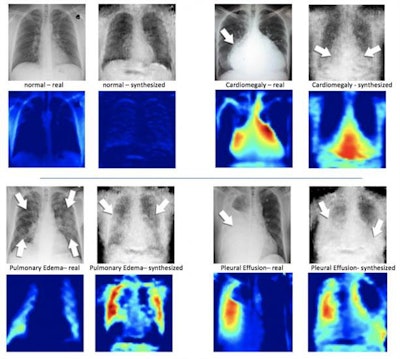
Algorithms based on generative adversarial networks are made up of two networks: one that generates images and another that tries to discriminate the artificial images from real ones. Both networks are trained to the point where the discriminator network can no longer tell real from artificial images -- when this happens, the synthesized images are ready for incorporation into a database with real images.
"This provides a greater quantity of data for training and improves the performance of these systems in identifying rare conditions," Valaee said.
To prove their algorithm, the MIMLab researchers compared the accuracy of their AI system when analyzing the augmented database with an original dataset. They found that the interpretation algorithm saw its accuracy improve by 20% for common conditions and up to 40% for some pathologies.
Another advantage of synthesized images is that they can be shared with researchers without fear of violating patient privacy, which can be an issue with real images.
"It's exciting because we've been able to overcome a hurdle in applying artificial intelligence to medicine by showing that these augmented datasets help to improve classification accuracy," Valaee said. "Deep learning only works if the volume of training data is large enough, and this is one way to ensure we have neural networks that can classify images with high precision."