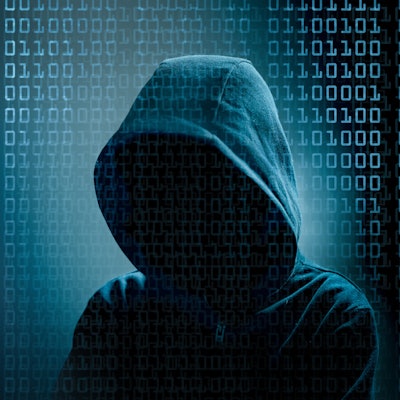
Artificial intelligence (AI) algorithms in healthcare -- including those used in medical imaging -- are vulnerable to being manipulated by users to produce inaccurate results, and now is the time to consider how to defend against these adversarial attacks, according to an article published March 22 in Science.
Adversarial attacks involve users applying a small change in how inputs are presented to an algorithm to completely alter its output and confidently deliver wrong conclusions, wrote a team of researchers led by Samuel Finlayson of Harvard Medical School. After reviewing the motivations for various players in the healthcare system to use adversarial attacks on AI algorithms, the researchers called for medical, technical, legal, and ethical experts to be actively engaged in addressing these potential threats.
While cutting-edge adversarial attacks have yet to be found in healthcare AI technology, less-formalized adversarial practice is extremely common in areas such as medical billing. This includes rampant, intentional "upcoding" for procedures, as well as making subtle billing code adjustments aimed at avoiding denial of coverage, according to the researchers (Science, Vol. 363:6433, pp. 1287-1289).
With the rapid pace of AI technology development, it's challenging to determine if the problem of adversarial attacks should be addressed now or later, when algorithms and the protocols governing their use have been firmed up, according to the researchers.
"At best, acting now could equip us with more resilient systems and procedures, heading the problem off at the pass," they wrote. "At worst, it could lock us into inaccurate threat models and unwieldy regulatory structures, stalling developmental progress and robbing systems of the flexibility they need to confront unforeseen threats."
Given sufficient political and institutional will, some incremental defensive steps could be taken, however. This could include amending or extending best practices in hospital labs -- already enforced in the U.S. through regulatory measures -- to also encompass best practices for protecting against adversarial attacks.
"For example, in situations in which tampering with clinical data or images might be possible, a 'fingerprint' hash of the data might be extracted and stored at the moment of capture," they wrote.
To determine if data have been tampered with or changed after acquisition, investigators could then compare the original "hash" with what was fed through an algorithm.
"Such an intervention would rely on a health IT infrastructure capable of supporting the capture and secure storage of these hashes," they wrote. "But as a strictly regulated field with a focus on accountability and standards of procedure, healthcare may be very well suited to such adaptations."
The rapid proliferation of vulnerable algorithms and strong motives to manipulate these algorithms make healthcare a plausible ground zero for adversarial examples to enter real-world practice, according to the authors.
"A clear-eyed and principled approach to adversarial attacks in the healthcare context -- one which builds the groundwork for resilience without crippling rollout and sets ethical and legal standards for line-crossing behavior -- could serve as a critical model for these future efforts," they wrote.