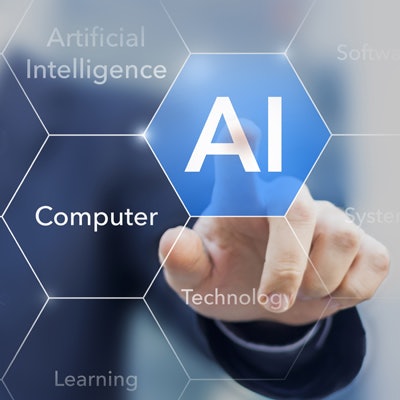
The rapid uptake of artificial intelligence (AI) and deep learning in healthcare is being driven by seven factors, including an increasingly crucial role for digital imaging in medicine, according to one of two Viewpoint articles published online August 30 in the Journal of the American Medical Association.
In the first Viewpoint article, Geoffrey Hinton, PhD, of the Google Brain Team and the University of Toronto explained the technology behind AI and deep learning. In the second article, Dr. C. David Naylor of the University of Toronto focused on seven key factors spurring the adoption of AI and deep learning:
-
Digital imaging in all of its forms is becoming more powerful and more integral to medicine and healthcare.
Deep learning can capitalize on all of the patterns that can be extracted from very large datasets and used for interpreting still and moving images, according to Naylor.
"Deep learning and related machine-learning methods can also learn from massively greater numbers of images than any human expert, continue learning and adapting over time, mitigate interobserver variability, and facilitate better decision-making and more effective image-guided therapy," he wrote.
- Digitization of health-related records is accelerating, as is sharing of high-quality fully labeled and specialized datasets.
- Deep learning is highly adaptable for integrative analysis of heterogeneous datasets assembled from diverse sources.
- Deep learning has enormous capacity to inform the process of discovery in health research and to facilitate hypothesis generation by identifying novel associations.
- Deep learning shows promise for streamlining routine work by healthcare professionals and empowering patients, thereby promoting a safer, more humane, and participatory paradigm for healthcare.
- Deep learning is diffusing rapidly through a combination of open-source and proprietary programs.
- None of these developments depend on improvements in the basic technology of deep learning.
Naylor noted that deep learning also exemplifies a broader trend toward the convergence of health and data sciences.
"Barriers to adoption will rightly be more rigid in healthcare than in many other fields in which software programs relying on deep learning and other forms of machine learning are used daily by billions of people," he wrote. "However, pressure to deploy deep learning and a range of tools derived from modern data science will be relentless, given the extraordinarily rich information now available to characterize and follow vast numbers of patients, the ongoing challenges of making sense of the complexity of human biology and healthcare systems, and the potential for smart information technology to support tomorrow's clinicians in the provision of safe, effective, efficient, and humanistic care."
In an accompanying editorial, Dr. William Stead of Vanderbilt University Medical Center wrote that AI and deep learning are now entering the mainstream of clinical medicine.
"This technology can augment human intelligence to improve decision-making and operational processes," Stead wrote. "Physicians need to actively engage to adapt their practice and to shape the technology."