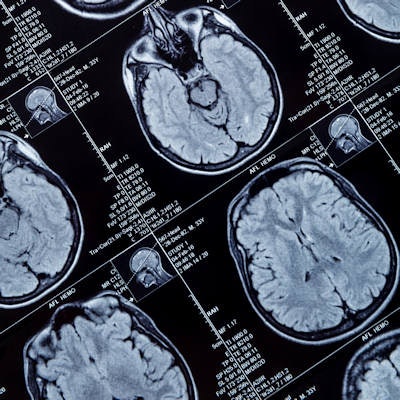
An artificial intelligence (AI) algorithm that analyzed free text in patient reports was better than existing risk models in predicting which symptomatic individuals were actually experiencing a transient ischemic attack (TIA), according to research published online January 17 in Stroke.
Australian researchers trained and tested a number of deep-learning models to predict the cause of TIA-like symptoms via natural language processing (NLP) of the free-text descriptions of the patient's complaint history. They found that the best-performing model -- a convolutional neural network (CNN) -- yielded its highest level of accuracy once MRI reports were added to the training data.
"Deep learning-based natural language processing, in particular convolutional neural networks, based on medical free-text, may prove effective in prediction of the cause of TIA-like presentations," wrote the researchers led by Dr. Stephen Bacchi of the Royal Adelaide Hospital.
Triaging challenges
Prior studies have shown that timely treatment after a TIA -- a temporary stroke-like attack -- can dramatically reduce the risk of early recurrent stroke. However, many of the patients referred to TIA clinics haven't actually experienced a TIA. As a result, prioritizing individuals who do have a cerebrovascular cause of their symptoms is important for triaging purposes, the researchers noted.
"Although recognition tools and risk scores, such as the ABCD2 score and Dawson TIA recognition score, exist, their precision is imperfect, and real-world implementation of these tools may be suboptimal, particularly in primary care settings," they wrote.
To address the ongoing clinical need for methods of triaging referrals to TIA clinics, the researchers sought to determine if deep learning could provide an automated method to help predict the likelihood of a cerebrovascular cause of the patient's presenting symptoms. They first gathered a variety of data for consecutive patients seen in neurovascular TIA clinics from two tertiary hospitals in the state of South Australia from 2012 to 2018.
Four deep-learning models were initially trained only with the treating vascular neurologist's final diagnosis and the patient's history of presenting complaint -- typically entered by TIA nurses and reviewed by neurovascular consultants. In testing, the CNN was found to produce the highest area under the curve (AUC).
The researchers evaluated the effect of incorporating additional information for training such as past medical history, medications, head CT reports, and brain MRI reports. They found that the CNN's best performance came after MRI reports alone were added to the CNN's training data.
Performance of CNN for predicting cause of TIA-like presentation | |||
ABCD2 score | CNN trained only with histories of presenting complaint | CNN trained with addition of MRI reports | |
AUC | 0.674 | 0.81 | 0.88 |
The CNN also offered significantly superior performance to the ABCD2 score, a common stroke risk-prediction method that produced an AUC of only 0.674. The researchers noted that trained deep learning-based NLP models aren't computationally taxing and could be used to provide automated classification of individual referrals on a regular computer.
Future work
Future research in this area may include applying deep learning-based natural language processing to the prediction of stroke risk after a transient ischemic attack, according to the researchers.
"Similar applications of [deep learning-based] NLP, in terms of diagnostic prediction and potential triage of clinic referrals, could be explored in other specialty areas with high referral numbers," they wrote.
The technique now requires validation in larger datasets, multiple centers, and prospective primary care referrals, as well as investigation of its efficacy in predicting recurrent stroke, according to the group.